How to have a healthy relationship with tech
∞ Sep 10, 2018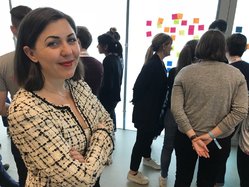
At Well+Good, the wonderful Liza Kindred describes how to make personal technology serve you, instead of the reverse. It all starts with realizing that your inability to put down your phone isn’t a personal failing, it’s something that’s been done to you:
“The biggest problem with how people engage with technology is technology, not the people,” she says. “Our devices and favorite apps are all designed to keep us coming back for more. That being said, there are many ways for us to intervene in our own relationships with tech, so that we can live this aspect of our lives in a way we can be proud of.”
Liza offers several pointers for putting personal technology in its place. My personal favorite:
Her biggest recommendation is turning off all notifications not sent by a human. See ya, breaking news, Insta likes, and emails. “Your time is more valuable than that,” Kindred says.
Alas, these strategies are akin to learning self-defense skills during a crime wave. They’re helpful (critical, even), but the core problem remains. In this case, the “crime wave” is the cynical, engagement-hungry strategies that too many companies employ to keep people clicking and tapping. And clicking and tapping. And clicking and tapping.
Liza’s on the case there, too. Her company Holy Shift helps people find mindful and healthy experiences in a modern, distracting, engagement-heavy world. I’ve participated in her Mindful Technology workshops and they’re mind opening. Liza demonstrates that design patterns and business models that you might take for granted as a best practice do more damage than you realize.
Meanwhile, we’ll have to continue to sharpen those self-defense skills.
“Trigger for a rant”
∞ Jul 1, 2018In his excellent Four Short Links daily feature, Nat Torkington has something to say about innovation poseurs—in the mattress industry:
Why So Many Online Mattress Brands – trigger for a rant: software is eating everything, but that doesn’t make everything an innovative company. If you’re applying the online sales playbook to product X (kombucha, mattresses, yoga mats) it doesn’t make you a Level 9 game-changing disruptive TechCo, it makes you a retail business keeping up with the times. I’m curious where the next interesting bits of tech are.
Should computers serve humans, or should humans serve computers?
∞ Jun 30, 2018Nolan Lawson considers dystopian and utopian possibilities for the future, with a gentle suggestion that front-line technologists have some agency here. What kind of world do you want to help build?
The core question we technologists should be asking ourselves is: do we want to live in a world where computers serve humans, or where humans serve computers?
Or to put it another way: do we want to live in a world where the users of technology are in control of their devices? Or do we want to live in a world where the owners of technology use it as yet another means of control over those without the resources, the knowledge, or the privilege to fight back?
s5e11: Things That Have Caught My Attention
∞ May 20, 2018In a recent edition of his excellent stream-of-consciousness newsletter, Dan Hon considers Alexa Kids Edition in which, among other things, Alexa encourages kids to say “please.” There are challenges and pitfalls, Dan writes, in designing a one-size-fits-all system that talks to children and, especially, teaches them new behaviors.
Parenting is a very personal subject. As I have become a parent, I have discovered (and validated through experimental data) that parents have very specific views about how to do things! Many parents do not agree with each other! Parents who agree with each other on some things do not agree on other things! In families where there are two parents there is much scope for disagreement on both desired outcome and method!
All of which is to say is that the current design, architecture and strategy of Alexa for Kids indicates one sort of one-size-fits-all method and that there’s not much room for parental customization. This isn’t to say that Amazon are actively preventing it and might not add it down the line - it’s just that it doesn’t really exist right now. Honan’s got a great point that:
"[For example,] take the magic word we mentioned earlier. There is no universal norm when it comes to whatâs polite or rude. Manners vary by family, culture, and even region. While âyes, sirâ may be de rigueur in Alabama, for example, it might be viewed as an element of the patriarchy in parts of California."
AI Is Harder Than You Think
∞ May 20, 2018In the New York Times opinion section, Gary Marcus and Ernest Davis suggest that today’s data-crunching model for artificial intelligence is not panning out. Instead of truly understanding logic or language, today’s machine learning instead identifies data patterns to recognize and reflect human behavior. The systems this approach creates tends to mimic more than think. As a result, we have some impressive but incredibly narrow applications of AI. The culmination of artificial intelligence appears to be making salon appointments.
Decades ago, the approach was different. The AI field tried to understand the elements of human thought—and teach machines to actually think. The goal proved elusive and the field drifted instead to what machines were already better at understanding, pattern recognition. Marcus and Davis say the detour has not proved helpful:
Once upon a time, before the fashionable rise of machine learning and “big data,” A.I. researchers tried to understand how complex knowledge could be encoded and processed in computers. This project, known as knowledge engineering, aimed not to create programs that would detect statistical patterns in huge data sets but to formalize, in a system of rules, the fundamental elements of human understanding, so that those rules could be applied in computer programs. Rather than merely imitating the results of our thinking, machines would actually share some of our core cognitive abilities.
That job proved difficult and was never finished. But “difficult and unfinished” doesn’t mean misguided. A.I. researchers need to return to that project sooner rather than later, ideally enlisting the help of cognitive psychologists who study the question of how human cognition manages to be endlessly flexible.
Today’s dominant approach to A.I. has not worked out. Yes, some remarkable applications have been built from it, including Google Translate and Google Duplex. But the limitations of these applications as a form of intelligence should be a wake-up call. If machine learning and big data can’t get us any further than a restaurant reservation, even in the hands of the world’s most capable A.I. company, it is time to reconsider that strategy.
Google Duplicitous
∞ May 9, 2018Jeremy Keith comments on Google’s announcement of Google Duplex:
The visionaries of technology—Douglas Engelbart, J.C.R Licklider—have always recognised the potential for computers to augment humanity, to be bicycles for the mind. I think they would be horrified to see the increasing trend of using humans to augment computers.
Do You Have “Advantage Blindness”?
∞ Apr 27, 2018At Harvard Business Review, Ben Fuchs, Megan Reitz, and John Higgins consider the responsibility of identifying our own blind spots—the biases, privileges, and disadvantages we haven’t admitted to ourselves. It’s important (and sometimes bruising) work—all the more important if you’re in a privileged position that gives you the leverage to make a difference for others.
To address inequality of opportunity, we need to acknowledge and address the systemic advantages and disadvantages that people experience daily. For leaders, recognizing their advantage blindness can help to reduce the impact of bias and create a more level playing field for everyone. Being advantaged through race and gender come with a responsibility to do something about changing a system that unfairly disadvantages others.
The Juvet Agenda
∞ Oct 30, 2017I had the privilege last month of joining 19 other designers, researchers, and writers to consider the future (both near and far) of artificial intelligence and machine learning. We headed into the woods—to the Juvet nature retreat in Norway—for several days of hard thinking. Under the northern lights, we considered the challenges and opportunities that AI presents for society, for business, for our craft—and for all of us individually.
Answers were elusive, but questions were plenty. We decided to share those questions, and the result is the Juvet Agenda. The agenda lays out the urgent themes surrounding AI‚and presents a set of provocations for teasing out a future we want to live in:
Artificial intelligence? It’s complicated. It’s the here and now of hyper-efficient algorithms, but it’s also the heady possibility of sentient systems. It might be history’s greatest opportunity or its worst existential threat — or maybe it will only optimize what we’ve already got. Whatever it is and whatever it might become, the thing is moving too fast for any of us to sit still. AI demands that we rethink our methods, our business models, maybe even our cultures.
In September 2017, 20 designers, urbanists, researchers, writers, and futurists gathered at the Juvet nature retreat among the fjords and forests of Norway. We came together to consider AI from a humanist perspective, to step outside the engineering perspective that dominates the field. Could we sort out AI’s contradictions? Could we describe its trajectory? Could we come to any conclusions?
Across three intense days the group captured ideas, played games, drew diagrams, and snapped photos. In the end, we arrived at more questions than answers — and Big Questions at that. These are not topics we can or should address alone, so we share them here.
Together these questions ask how we can shape AI for a world we want to live in. If we don’t decide for ourselves what that world looks like, the technology will decide for us. The future should not be self-driving; let’s steer the course together.
Stop Pretending You Really Know What AI Is
∞ Sep 9, 2017“Artificial intelligence” is broadly used in everything from science fiction to the marketing of mundane consumer goods, and it no longer has much practical meaning, bemoans John Pavlus at Quartz. He surveys practitioners about what the phrase does and doesn’t mean:
It’s just a suitcase word enclosing a foggy constellation of “things”—plural—that do have real definitions and edges to them. All the other stuff you hear about—machine learning, deep learning, neural networks, what have you—are much more precise names for the various scientific, mathematical, and engineering methods that people employ within the field of AI.
But what’s so terrible about using the phrase “artificial intelligence” to enclose all that confusing detail—especially for all us non-PhDs? The words “artificial” and “intelligent” sound soothingly commonsensical when put together. But in practice, the phrase has an uncanny almost-meaning that sucks adjacent ideas and images into its orbit and spaghettifies them.
Me, I prefer to use “machine learning” for most of the algorithmic software I see and work with, but “AI” is definitely a convenient (if overused) shorthand.
AI Guesses Whether You're Gay or Straight from a Photo
∞ Sep 9, 2017Well this seems ominous. The Guardian reports:
Artificial intelligence can accurately guess whether people are gay or straight based on photos of their faces, according to new research that suggests machines can have significantly better “gaydar” than humans.
The study from Stanford University – which found that a computer algorithm could correctly distinguish between gay and straight men 81% of the time, and 74% for women – has raised questions about the biological origins of sexual orientation, the ethics of facial-detection technology, and the potential for this kind of software to violate people’s privacy or be abused for anti-LGBT purposes.
The Pop-Up Employer: Build a Team, Do the Job, Say Goodbye
∞ Aug 2, 2017Big Medium is what my friend and collaborator Dan Mall calls a design collaborative. Dan runs his studio Superfriendly the same way I run Big Medium: rather than carry a full-time staff, we both spin up bespoke teams from a tight-knit network of well-known domain experts. Those teams are carefully chosen to meet the specific demands of each project. It’s a very human, very personal way to source project teams.
And so I was both intrigued and skeptical to read about an automated system designed to do just that at a far larger scale. Noam Scheiber reporting for The New York Times:
True Story was a case study in what two Stanford professors call “flash organizations” — ephemeral setups to execute a single, complex project in ways traditionally associated with corporations, nonprofit groups or governments. […]
And, in fact, intermediaries are already springing up across industries like software and pharmaceuticals to assemble such organizations. They rely heavily on data and algorithms to determine which workers are best suited to one another, and also on decidedly lower-tech innovations, like middle management. […]
“One of our animating goals for the project was, would it be possible for someone to summon an entire organization for something you wanted to do with just a click?” Mr. Bernstein said.
The fascinating question here is how systems might develop algorithmic proxies for the measures of trust, experience, and quality that weave the fabric of our professional networks. But even more intriguing: how might such models help to connect underrepresented groups with work they might otherwise never have access to? For that matter, how might those models introduce me to designers outside my circle who might introduce more diverse perspectives into my own work?
The BBQ and the Errant Butler
∞ Aug 2, 2017Marek Pawlowski shares a tale of a dinner party taken hostage by a boorish Alexa hell-bent on selling the guests music.
Amid the flashy marketing campaigns and rapid technological advances surrounding virtual assistants like Alexa, Cortana and Siri, few end users seem willing to question how the motivation of their creators is likely to affect the overall experience. Amazon has done much to make Alexa smart, cheap and useful. However, it has done so in service of an over-arching purpose: retailing. Of course, Google, Microsoft and Apple have ulterior motives for their own assistants, but it should come as no surprise that Alexa is easily sidetracked by her desire to sell you things.
Toy Story Lessons for the Internet of Things
∞ Aug 2, 2017Dan Gärdenfors ponders how to handle “leadership conflicts” in IoT devices:
In future smart homes, many interactions will be complex and involve combinations of different devices. People will need to know not only what goes on but also why. For example, when smart lights, blinds and indoor climate systems adjust automatically, home owners should be able to know what triggered it. Was it weather forecast data or the behaviour of people at home that made the thermostat lower the temperature? Which device made the decision and told the others to react? Especially when things donât end up the way we want them to, smart objects need to communicate more, not less.
As we introduce more sensors, services, and smart gadgets into our life, some of them will inevitably collide. Which one “wins”? And how do we as users see the winner (or even understand that there was a conflict in the first place)?
UX design gets complicated when you introduce multiple triggers from multiple opinionated systems. And of course all those opinionated systems should bow to the most important opinion of all: the user’s. But even that is complicated in a smart-home environment where there are multiple users who have changing needs, desires, and contexts throughout the day. Fun!
Politics Are a Design Constraint
∞ Aug 2, 2017Designers, if you believe that politics don’t belong at work, guess what: your work is itself political. Software channels behavior, and that means that it’s freighted with values.
Ask yourself: as a designer, what are the behaviors I’m shaping, for what audience, to what end, and for whose benefit? Those questions point up the fact that software is ideological. The least you can do is own that fact and make sure that your software’s politics line up with your own. John Warren Hanawalt explains why:
Designers have a professional responsibility to consider what impact their work has—whether the project is explicitly “political” or not. Design can empower or disenfranchise people through the layout of ballots or UX of social network privacy settings.
Whose voices are amplified or excluded by the platforms we build, who profits from or is exploited by the service apps we code, whether we have created space for self-expression or avenues for abuse: these are all political design considerations because they decide who is represented, who can participate and at what cost, and who has power. […]
If you’re a socially conscious designer, you don’t need to quit your job; you need to do it. That means designing solutions that benefit people without marginalizing or harming others. When your boss or client asks you to do something that might do harm, you have to say no. And if you see unethical behavior happening in other areas of your company, fight for something better. If you find a problem, you have a problem. Good thing solving problems is your job.
AI Firstâwith UX
∞ Aug 2, 2017When mobile exploded a decade ago, many of us wrestled with designing for the new context of freshly portable interfaces. In fact, we often became blinded by that context, assuming that mobile interfaces should be optimized strictly for on-the-go users: we overdialed on location-based interactions, short attention spans, micro-tasks. The “lite” mobile version ruled.
It turned out that the physical contexts of mobile gadgetsâdevice and environmentâwere largely red herrings. The notion of a single “mobile context” was a myth that distracted from the more meaningful range of “softer” contexts these devices introduced by unchaining us from the desktop. The truth was that we now had to design for a huge swath of temporal, behavioral, emotional, and social contexts. When digital interfaces can penetrate any moment of our lives, the designer can no longer assume any single context in which it will be used.
This already challenging contextual landscape is even more complicated for predictive AI assistants that constantly run in the background looking for moments to provide just-in-time info. How much do they need to know about current context to judge the right moment to interrupt with (hopefully) useful information?
In an essay for O’Reilly, Mike Loukides explores that question, concluding that it’s less a concern of algorithm design than of UX design:
What’s the experience I want in being âassistedâ How is that experience designed? A design that requires me to expend more effort to take advantage of the assistant’s capabilities is a step backward.
The design problem becomes more complex when we think about how assistance is delivered. Norvig’s "reminders" are frequently delivered in the form of asynchronous notifications. That’s a problem: with many applications running on every device, users are subjected to a constant cacophony of notifications. Will AI be smart enough to know what notifications are actually wanted, and which are just annoyances? A reminder to buy milk? That’s one thing. But on any day, there are probably a dozen or so things I need, or could possibly use, if I have time to go to the store. You and I probably don’t want reminders about all of them. And when do we want these reminders? When we’re driving by a supermarket, on the way to the aforementioned doctor’s appointment? Or would it just order it from Amazon? If so, does it need your permission? Those are all UX questions, not AI questions.
We’ve made lots of fast progress in just the last few yearsâmonths, evenâin crafting remarkably accurate algorithms. We’re still getting started, though, in crafting the experiences we wrap around them. There’s lots of work to be done right now by designers, including UX research at unprecedented scale, to understand how to put machine learning to use as design material. I have ideas and design principles about how to get started. In the meantime, I really like the way Mike frames the problem:
In a future where humans and computers are increasingly in the loop together, understanding context is essential. But the context problem isn’t solved by more AI. The context is the user experience. What we really need to understand, and what we’ve been learning all too slowly for the past 30 years, is that technology is the easy part.
Making Software with Casual Intelligence
∞ Aug 2, 2017The most broadly impactful technologies tend to be the ones that become mundane—cheap, expected, part of the fabric of everyday life. We absorb them into our lives, their presence assumed, their costs negligible. Electricity, phones, televisions, internet, refrigeration, remote controls, power windows—once-remarkable technologies that now quietly improve our lives.
That’s why the aspects of machine learning that excite me most right now are the small and mundane interventions that designers and developers can deploy today in everyday projects. As I wrote in Design in the Era of the Algorithm, there are so many excellent (and free!) machine-learning APIs just waiting to be integrated into our digital products. Machine learning is the new design material, and it’s ready today, even for the most modest product features.
All of this reminds me of an essay my friend Evan Prodromou wrote last year about making software with casual intelligence. It’s a wonderful call to action for designers and developers to start integrating machine learning into everyday design projects.
Programmers in the next decade are going to make huge strides in applying artificial intelligence techniques to software development. But those advances aren’t all going to be in moonshot projects like self-driving cars and voice-operated services. They’re going to be billions of incremental intelligent updates to our interfaces and back-end systems.
I call this _casual intelligence_ — making everything we do a little smarter, and making all of our software that much easier and more useful. It’s casual because it makes the user’s experience less stressful, calmer, more leisurely. It’s also casual because the developer or designer doesn’t think twice about using AI techniques. Intelligence becomes part of the practice of software creation.
Evan touches on one of the most intriguing implications of designing data-driven interfaces. When machines generate both content and interaction, they will often create experiences that designers didn’t imagine (both for better and for worse). The designer’s role may evolve into one of corralling the experience in broad directions, rather than down narrow paths. (See conversational interfaces and open-ended, Alexa/Siri-style interactions, for example.)
Designers need to stop thinking in terms of either-or interfaces — either we do it this way, or we do it that way. Casual intelligence lets interfaces become _and-also_ — different users have different experiences. Some users will have experiences never dreamed of in your wireframes — and those may be the best ones of all.
In the AI Age, “Being Smart” Will Mean Something Completely Different
∞ Aug 2, 2017As machines become better than people at so many things, the natural question is what’s left for humans—and indeed what makes us human in the first place? Or more practically: what is the future of work for humans if machines are smarter than us in so many ways? Writing for Harvard Business Review, Ed Hess suggests that the answer is in shifting the meaning of human smarts away from information recall, pattern-matching, fast learning—and even accuracy.
What is needed is a new definition of being smart, one that promotes higher levels of human thinking and emotional engagement. The new smart will be determined not by what or how you know but by the quality of your thinking, listening, relating, collaborating, and learning. Quantity is replaced by quality. And that shift will enable us to focus on the hard work of taking our cognitive and emotional skills to a much higher level.
We will spend more time training to be open-minded and learning to update our beliefs in response to new data. We will practice adjusting after our mistakes, and we will invest more in the skills traditionally associated with emotional intelligence. The new smart will be about trying to overcome the two big inhibitors of critical thinking and team collaboration: our ego and our fears. Doing so will make it easier to perceive reality as it is, rather than as we wish it to be. In short, we will embrace humility. That is how we humans will add value in a world of smart technology.
In a Few Years, No Investors Are Going To Be Looking for AI Startups
∞ Jul 9, 2017Frank Chen of Andreessen Horowitz suggests that while machine learning and AI are today’s new hotness, they’re bound to be the humdrum norm in just a few short years. Products that don’t have it baked in will seem oddly quaint:
Not having state-of-the-art AI techniques powering their software would be like not having a relational database in their tech stack in 1980 or not having a rich Windows client in 1987 or not having a Web-based front end in 1995 or not being cloud native in 2004 or not having a mobile app in 2009. In other words, in a small handful of years, software without AI will be unthinkable.
So ambitious founders will need to invest some other way to differentiate themselves from the crowd — and investors will be looking for other ways to decide whether to fund a startup. And investors will stop looking for AI-powered startups in exactly the same way they don’t look for database-inside or cloud-native or mobile-first startups anymore. All those things are just assumed.
As Chen says, this feels like mobile just a few years ago. Just as mobile was the oxygen feeding emerging interactions and capabilities, machine learning is doing the same now. All the new interactions, all the new digital superpowers, they’re all being fueled by machine learning and algorithms.
In 2012, I wrote a chapter for The Mobile Book, for which Jeremy Keith wrote a prescient foreword. “This book is an artefact of its time,” he wrote. “There will come a time when this book will no longer be necessary, when designing and developing for mobile will simply be part and parcel of every Web worker’s lot.”
Yep, five years later, mobile is an assumed part of the job. If you were writing a “Machine Learning Book” today, you could borrow the same observation for the foreword. It’s time to get your game on now, since this will be an assumed capability in short order.
If you’re a designer wondering how you fit into all of this, I have some ideas: Design in the era of the algorithm.