Beware of Botshit
∞ Oct 13, 2024botshit noun: hallucinated chatbot content that is uncritically used by a human for communication and decision-making tasks. “The company withdrew the whitepaper due to excessive botshit, after the authors relied on unverified machine-generated research summaries.”
From this academic paper on managing the risks of using generated content to perform tasks:
Generative chatbots do this work by ‘predicting’ responses rather than ‘knowing’ the meaning of their responses. This means chatbots can produce coherent sounding but inaccurate or fabricated content, referred to as ‘hallucinations’. When humans use this untruthful content for tasks, it becomes what we call ‘botshit’.
See also: slop.
A Radically Adaptive World Model
∞ Oct 13, 2024Ethan Mollick posted this nifty little demo of a research project that generates a world based on Counter-Strike, frame by frame in response to your actions. What’s around that corner at the end of the street? Nothing, that portion of the world hasn’t been created yet—until you turn in that direction, and the world is created just for you in that moment.
This is not a post that proposes the future of gaming or that tech will replace well-crafted game worlds and the people who make them. This proof of concept is nowhere near ready or good enough for that, except perhaps as a tool to assist/support game authors.
Instead, it’s interesting as a remarkable example of a radically adaptive interface, a core aspect of Sentient Design experiences. The demo and the research paper behind it show a whole world being conceived, compiled, and delivered in real time. What happens when you apply this thinking to a web experience? To a data dashboard? To a chat interface? To a calculator app that lets you turn a blank canvas into a one-of-a-kind on-demand interface?
The risk of radically adaptive interfaces is that they turn into robot fever dreams without shape or destination. That’s where design comes in: to conceive and apply thoughtful constraints and guardrails. It’s weird and hairy and different from what’s come before.
Far from replacing designers (or game creators), these experiences require designers more than ever. But we have to learn some new skills and point them in new directions.
Exploring the AI Solution Space
∞ Oct 13, 2024Jorge Arango explores what it means for machine intelligence to be “used well” and, in particular, questions the current fascination with general-purpose, open-ended chat interfaces.
There are obvious challenges here. For one, this is the first time weâve interacted with systems that match our linguistic abilities while lacking other attributes of intelligence: consciousness, theory of mind, pride, shame, common sense, etc. AIsâ eloquence tricks us into accepting their output when we have no competence to do so.
The AI-written contract may be better than a human-written one. But can you trust it? After all, if youâre not a lawyer, you donât know what you donât know. And the fact that the AI contract looks so similar to a human one makes it easy for you to take its provenance for granted. That is, the better the outcome looks to your non-specialist eyes, the more likely you are to give up your agency.
Another challenge is that ChatGPTâs success has driven many people to equate AIs with chatbots. As a result, the current default approach to adding AI to products entails awkwardly grafting chat onto existing experiences, either for augmenting search (possibly good) or replacing human service agents (generally bad.)
But these âchatbotâ scenarios only cover a portion of the possibility space â and not even the most interesting one.
I’m grateful for the call to action to think beyond chat and general-purpose, open-ended interfaces. Those have their place, but there’s so much more to explore here.
The popular imagination has equated intelligence with convincing conversation since Alan Turing proposed his “imitation game” in 1950. The concept is simple: if a system can fool you into thinking you’re talking to a human, it can be considered intelligent. For the better part of a century, the Turing Test has shaped popular expectations of machine intelligence from science fiction to Silicon Valley. Chat is an interaction cliché for AI that we have to escape (or at least question), but it has a powerful gravitational force. “Speaks well = thinks well” is a hard perception to break. We fall for it with people, too.
The “AI can make mistakes” labels don’t cut it.
Given the outsized trust we have in systems that speak so confidently, designers have a big challenge when crafting intelligent interfaces: how can you engage the user’s agency and judgment when the answer is not actually as confident as the LLM delivers it? Communicating the accuracy/confidence of results is a design job. The “AI can make mistakes” labels don’t cut it.
This isn’t a new challenge. I’ve been writing about systems smart enough to know they’re not smart enough for years. But the problem gets steeper as the systems appear outwardly smarter and lull us into false confidence.
Jorge’s 2x2 matrix of AI control vs AI accuracy is a helpful tool to at least consider the risks as you explore solutions.
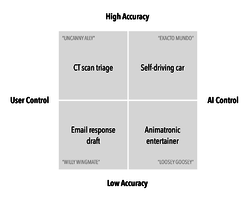
This is a tricky time. It’s natural to seek grounding in times of change, which can cause us to cling too tightly to assumptions or established patterns. Loyalty to the long-held idea that conflates conversation with intelligence is doing a disservice. Conversation between human and machine doesnât have to mean literal dialogue. Letâs be far more expansive in what we consider âchatâ and unpack the broad forms these interactions can take.
Introducing Generative Canvas
∞ Oct 8, 2024On-demand UI! Salesforce announced its pilot of “generative canvas,” a radically adaptive interface for CRM users. It’s a dynamically generated dashboard that uses AI to assemble the right content and UI elements based on your specific context or request. Look out, enterprise, here comes Sentient Design.
I love to see big players doing this. Here at Big Medium, we’re building on similar foundations to help our clients build their own AI-powered interfaces. It’s exciting stuff! Sentient Design is about creating AI-mediated experiences that are aware of context/intent so that they can adapt in real time to specific needs. Veronika Kindred and I call these radically adaptive interfaces, and it shows that machine-intelligent experiences can be so much more than chat. This new Salesforce experience offers a good example.
For Salesforce, generative canvas is an intelligent interface that animates traditional UI in new and effective ways. It’s a perfect example of a first-stage radically adaptive interface—and one that’s well suited to the sturdy reliability of enterprise software. Generative canvas uses all of the same familiar data sources as a traditional Salesforce experience might, but it assembles and presents that data on the fly. Instead of relying on static templates built through a painstaking manual process, generative canvas is conceived and compiled in real time. That presentation is tailored to context: it pulls data from the user’s calendar to give suggested prompts and relevant information tailored to their needs. Every new prompt or new context gives you a new layout. (In Sentient Design’s triangle framework, we call this the Bespoke UI experience posture.)
So the benefits are: 1) highly tailored content and presentation to deliver the most relevant content in the most relevant format (better experience), and 2) elimination or reduction of manual configuration processes (efficiency).
In Sentient Design, we call this the Bespoke UI experience posture.
Never fear: you’re not turning your dashboard into a hallucinating robot fever dream. The UI stays on the rails by selecting from a collection of vetted components from Salesforce’s Lightning design system: tables, charts, trends, etc. AI provides radical adaptivity; the design system provides grounded consistency. The concept promises a stable set of data sources and design patterns—remixed into an experience that matches your needs in the moment.
This is a tidy example of what happens when you sprinkle machine intelligence onto a familiar traditional UI. It starts to dance and move. And this is just the beginning. Adding AI to the UX/UI layer lets you generate experiences, not just artifacts (images, text, etc.). And that can go beyond traditional UI to yield entirely new UX and interaction paradigms. That’s a big focus of Big Medium’s product work with clients these days—and of course of the Sentient Design book. Stay tuned, lots more to come.
Change Blindness
∞ Aug 13, 2024A great reminder from Ethan Mollick of how quickly things have changed in AI generation quality in the last 18 months. AI right now is the worst that it will ever be; only getting better from here. Good inspiration to keep cranking!
When I started this blog there were no AI chatbot assistants. Now, all indications that they are likely the fastest-adopted technology in recent history.
Plus, super cute otters.
Introducing Structured Outputs in the API
∞ Aug 7, 2024OpenAI introduced a bit of discipline to ensure that its GPT models are precise in the data format of their responses. Specifically, the new feature makes sure that, when asked, the model responds exactly to JSON schemas provided by developers.
Generating structured data from unstructured inputs is one of the core use cases for AI in today’s applications. Developers use the OpenAI API to build powerful assistants that have the ability to fetch data and answer questions via function calling(opens in a new window), extract structured data for data entry, and build multi-step agentic workflows that allow LLMs to take actions. Developers have long been working around the limitations of LLMs in this area via open source tooling, prompting, and retrying requests repeatedly to ensure that model outputs match the formats needed to interoperate with their systems. Structured Outputs solves this problem by constraining OpenAI models to match developer-supplied schemas and by training our models to better understand complicated schemas.
Most of us experience OpenAI’s GPT models as a chat interface, and that’s certainly the interaction of the moment. But LLMs are fluent in lots of languages—not just English or Chinese or Spanish, but JSON, SVG, Python, etc. One of their underappreciated talents is to move fluidly between different representations of ideas and concepts. Here specifically, they can translate messy English into structured JSON. This is what allows these systems to be interoperable with other systems, one of the three core attributes that define the form of AI-mediated experiences, as I describe in The Shape of Sentient Design.
What this means for product designers: As I shared in my Sentient Design talk, moving nimbly between structured and unstructured data is what enables LLMs to help drive radically adaptive interfaces. (This part of the talk offers an example.) This is the stuff that will animate the next generation of interaction design.
Alas, as in all things LLM, the models sometimes drift a bit from the specific ask—the JSON they come back with isn’t always what we asked for. This latest update is a promising direction for helping us get disciplined responses when we need it—so that Sentient Design experiences can reliably communicate with other systems.
Why I Finally Quit Spotify
∞ Aug 3, 2024In The New Yorker, Kyle Chayka bemoans the creeping blandness that settled into his Spotify listening experience as the company leaned into algorithmic personalization and playlists.
Issues with the listening technology create issues with the music itself; bombarded by generic suggestions and repeats of recent listening, listeners are being conditioned to rely on what Spotify feeds them rather than on what they seek out for themselves. “You’re giving them everything they think they love and it’s all homogenized,” Ford said, pointing to the algorithmic playlists that reorder tracklists, automatically play on shuffle, and add in new, similar songs. Listeners become alienated from their own tastes; when you never encounter things you don’t like, it’s harder to know what you really do.
This observation that the automation of your tastes can alienate you from them feels powerful. There’s obviously a useful and meaningful role for “more like this” recommendation and prediction engines. Still, there’s a risk when we overfit those models and eliminate personal agency and/or discovery in the experience. Surely there’s an opportunity to add more texture—a push and pull between lean-back personalization and more effortful exploration.
Let’s dial up the temperature on these models, or at least some of them. Instead of always presenting “more like this” recommendations, we could benefit from “more not like this,” too.
AI Is Confusing — Here’s Your Cheat Sheet
∞ Jul 28, 2024Scratching your head about diffusion models versus frontier models versus foundation models? Don’t know a token from a transformer? Jay Peters assembled a helpful glossary of AI terms for The Verge:
To help you better understand what’s going on, we’ve put together a list of some of the most common AI terms. We’ll do our best to explain what they mean and why they’re important.
Great, accessible resource for literacy in fundamental AI lingo.
Turning the Tables on AI
∞ Jul 28, 2024Oliver Reichenstein shares strategies for using AI to elevate your own writing instead of handing the job entirely to the robots. (This rhymes nicely with the core principle of Sentient Design: amplify judgment and agency instead of replacing it.)
Let’s turn the tables and have ChatGPT prompt us. Tell AI to ask you questions about what you’re writing. Push yourself to express in clear terms what you really want to say. Like this, for example:
I want to write [format] about [topic]. Ask me questions one at a time that force me to explain my idea.
Keep asking until your idea is clear to you.
Reichenstein is CEO and founder of iA, the maker of iA Writer. One of its features helps writers track facts and quotes from external sources. Reichenstein suggests using it to track AI-generated contributions:
What if the ChatGPT generated something useful that I want to keep? Paste it as a note Marked as AI. Use quotes, use markup, and note its origin. … iA Writer greys out text that you marked as AI so you can always discern what is yours and what isn’t.
It’s a good reminder that you can design personal workflows to use technology in ways that serve you best. What do you want AI to do for you? And as a product designer, how might you build this philosophy into your AI-mediated features?
Doctors Use A.I. Chatbots to Help Fight Insurance Denials
∞ Jul 28, 2024In The New York Times, Teddy Rosenbluth reports on doctors using AI tools to automate their fight with insurance companies’ (automated) efforts to refuse or delay payment:
Doctors and their staff spend an average of 12 hours a week submitting prior-authorization requests, a process widely considered burdensome and detrimental to patient health among physicians surveyed by the American Medical Association.
With the help of ChatGPT, Dr. Tward now types in a couple of sentences, describing the purpose of the letter and the types of scientific studies he wants referenced, and a draft is produced in seconds.
Then, he can tell the chatbot to make it four times longer. “If you’re going to put all kinds of barriers up for my patients, then when I fire back, I’m going to make it very time consuming,” he said.
I admire the dash of spite in this effort! But is this an example of tech leveling the playing field, or part of an AI-weaponized red-tape arms race that no one can win?
Still Trying to Sound Smart About AI? The Boss Is, Too
∞ Jul 28, 2024There’s a lot of “ready, fire, aim” in the industry right now as execs feel pressure to move on AI, even though most admit they don’t have confidence in how to use it. At The Wall Street Journal, Ray A. Smith rounds up some recent surveys that capture the situation:
Rarely has such a transformative, new technology spread and evolved so quickly, even before business leaders have grasped its basics.
No wonder that in a recent survey of 2,000 C-suite executives, 61% said AI would be a “game-changer.” Yet nearly the same share said they lacked confidence in their leadership teams’ AI skills or knowledge, according to staffing company Adecco and Oxford Economics, which conducted the survey.
The upshot: Many chief executives and other senior managers are talking a visionary game about AI’s promise to their staff—while trying to learn exactly what it can do.
Smith also points to a separate spring survey of 10,000 workers and executives that cited AI as a reason 71% of CEOs and two-thirds of other senior leaders said they had impostor syndrome in their positions.
With limited confidence at the top, AI innovation is trickling up from the bottom. (This rhymes with our strong belief at Big Medium that to be expert in a thing, you have to use the thing.)
In fact, much of what business leaders are gleaning about AI’s transformative potential is coming from individual employees, who are experimenting with AI on their own much faster than businesses are building bespoke, top-down applications of the technology, executives say.
In a survey of 31,000 working adults published by Microsoft last month, 75% of knowledge workers said they had started using AI on the job, the vast majority of whom reported bringing their own AI tools to work. Only 39% of the AI users said their employers had supplied them with AI training.
A Protopian Frontier
∞ Jun 28, 2024Take five minutes to watch A Protopian Future, an Ignite talk by Jenny Johnston. She offers a provocation to really think about and describe the future you imagine will come of the things you/we are trying so hard to change right now.
Here, Jenny asks what the world might look like 50 years after nuclear weapons are abolished. Your thing might be something different. You’re probably PUSHING for something to be changed / added / removed in the world; but what future are you PULLING toward? What’s the good and the bad—intentional or unintentional—of the future that you’re designing today?
Protopian stories imagine better futures but not perfect futures. They embrace a kind of messy progress. The reason we’re seeing this protopian surge right now is because humanity is in a weird place. We have this tangle of existential threats in front of us that we’re having a hard time seeing past and certainly our way through…. Protopian stories are powerful tools for reorienting ourselves toward hope and possibility and not dystopian dread.
After you watch Jenny’s video, go check out the farfutures.horizon2045.org project she edited. So great.
WWDC 2024: Apple Intelligence
∞ Jun 23, 2024John Gruber shares an under-reported tidbit from Apple’s many “Apple Intelligence” reveals:
The most unheralded aspect of Apple Intelligence is that the data centers Apple is building for Private Cloud Compute are not only carbon neutral, but are operating entirely on renewable energy sources. That’s extraordinary, and I believe unique in the entire industry.
LLMs are crazy-expensive across many dimensions, including environmental cost. Great to hear at least one company is tackling this head-on.
As usual, John also has lots of other insights on the announcements.
A Unified Theory of F*cks
∞ Jun 22, 2024The inimitable Mandy Brown reminds us that the f*cks we have to give are a limited resource. Spend them in the right place:
Why love your work? It won’t, of course, love you back. It can’t. Work isn’t a thing that can love. It isn’t alive, it isn’t and won’t ever be living. And my answer is: don’t. Don’t give a f*ck about your work. Give all your f*cks to the living. Give a f*ck about the people you work with, and the people who receive your work—the people who use the tools and products and systems or, more often than not, are used by them. Give a f*ck about the land and the sea, all the living things that are used or used up by the work, that are abandoned or displaced by it, or—if we’re lucky, if we’re persistent and brave and willing—are cared for through the work. Give a f*ck about yourself, about your own wild and tender spirit, about your peace and especially about your art. Give every last f*ck you have to living things with beating hearts and breathing lungs and open eyes, with chloroplasts and mycelia and water-seeking roots, with wings and hands and leaves. Give like every f*ck might be your last.
Because here’s what I’ve learned: if you give your f*cks to the unliving—if you plant those f*cks in institutions or systems or platforms or, gods forbid, interest rates—you will run out of f*cks.
Illuminate
∞ Jun 21, 2024Illuminate is an experimental project from Google that generates accessible, podcast-style interviews from academic papers:
Illuminate is an experimental technology that uses AI to adapt content to your learning preferences. Illuminate generates audio with two AI-generated voices in conversation, discussing the key points of select papers. Illuminate is currently optimized for published computer science academic papers.
The service has a waitlist, but you can try out some generated conversations (and I recommend that you do!). The enthusiasm, intonation, and ums & ahs are convincing and feel authentic to the genre that the project mimics. (See also the PDF to Podcast project which does similar things but with flatter voice results.)
But it’s not the seeming authenticity that feels important here. Machine-generated voices—even at this level of fidelity—are nothing new. What’s more interesting is how this project demonstrates what large language models (and now large multimodal models) are truly great at: they are prodigious translators and transformers of symbols, whether those symbols are for language, visuals, or broad concepts. These models can shift those symbols nimbly among formats: from English to Chinese to structured data to speech to UI components to audio to image. These are systems that can understand a concept they are given and then work their alchemy to present that concept in a new medium or language or format.
There are exciting opportunities here for unlocking content that is trapped in unfriendly formats (where the definition of “unfriendly” might be unique to the individual). This application leans into what generative AI is good at (understanding, transforming) around tightly scoped content—and avoids what these models are uneven at: answering questions or building content from scratch. How might this kind of transformation support education efforts, particularly around accessibility and inclusivity?
I Will F***ing Piledrive You If You Mention AI Again
∞ Jun 21, 2024Has breathless AI mania primed you for a ragey rant about overhyped technology and huckster pitchmen? Nikhil Suresh has you covered:
Most organizations cannot ship the most basic applications imaginable with any consistency, and you’re out here saying that the best way to remain competitive is to roll out experimental technology that is an order of magnitude more sophisticated than anything else your IT department runs, which you have no experience hiring for, when the organization has never used a GPU for anything other than junior engineers playing video games with their camera off during standup, and even if you do that all right there is a chance that the problem is simply unsolvable due to the characteristics of your data and business? This isn’t a recipe for disaster, it’s a cookbook for someone looking to prepare a twelve course f***ing catastrophe.
How about you remain competitive by fixing your shit?
There’s no such thing as a quick fix for a broken organization. And there’s no silver bullet for product excellence. AI is capable of amazing things, but you can’t shortcut great execution or ignore its very real downsides.
In another context, I often say, “High-performing teams have design systems, but having a design system won’t make you a high-performing team.” The same is true for AI.
There’s only one route to success: get your process and operations in order, understand the technologies you’re using, know their strengths and weaknesses, and above all: start with the right problem to solve.
We Need To Talk About Our AI Fetish
∞ Apr 21, 2024In a powerful and historically grounded essay, Jeremy Wagstaff asks that we not abdicate the vision for AI solely to the companies who stand to gain from it:
“Admittedly, it’s not easy to assess the implications of a complex technology like AI if you’re not an expert in it, so we tend to listen to the experts,” Wagstaff writes. “But listening to the experts should tell you all you need to know about the enormity of the commitment we’re making, and how they see the future of AI. And how they’re most definitely not the people we should be listening to.”
The potential impact of AI on work, culture, and individual agency is both deep and broad. And that impact will have effects that are both positive and negative—including effects that we haven’t yet imagined. We should be prepared to adapt to both, but history tells us that when policy is in the hands of those who would profit from transformative technology, bad things get buried. See oil, plastics, asbestos, pesticides, etc.—and now big tech, where Wagstaff points out we’ve seen a cynical evolution of how technology “helps” us:
At first Google search required us to define what it was that we wanted; Facebook et al required us to do define who and what we wanted to share our day with, and Twitter required us to be pithy, thoughtful, incisive, to debate. Tiktok just required us to scroll. At the end it turned out the whole social media thing was not about us creating and sharing wisdom, intelligent content, but for the platforms to outsource the expensive bit — creating entertainment — to those who would be willing to sell themselves, their lives, hawking crap or doing pratfalls.
AI has not reached that point. Yet. We’re in this early-Google summer where we have to think about what we want our technology to do for us. The search prompt would sit there awaiting us, cursor blinking, as it does for us in ChatGPT or Claude. But this is just a phase. Generative AI will soon anticipate what we want, or at least a bastardised version of what we want. It will deliver a lowest-common denominator version which, because it doesn’t require us to say it out loud, and so see in text see what a waste of our time we are dedicating to it, strip away while our ability to compute — to think — along with our ability, and desire, to do complex things for which we might be paid a salary or stock options.
It doesn’t have to turn out that way, of course. But it does require intention to change the course of technology and how companies and culture respectively profit from it, and not only financially. That intention has to come from many sources—from users, from policymakers, and from those of us who shape the digital experiences that use AI.
We all have to ask: What goals do we want to achieve with this technology? What is our vision for it? If we don’t decide for ourselves, the technology will decide for us. (Or the companies who would profit from it.) As I’m fond of saying: the future should not be self-driving.
Consider health care. What goals do we want to achieve by applying AI to patient care? If the primary goal is profit (reduce patient visit time and maximize the patient load), then the result might focus on AI taking over as much of the patient visit as possible. The machines would handle the intake, evaluate your symptoms and test, handle the diagnosis, suggest the course of action, and send you on your way. You might not even see another human being during most routine visits. If the experience ended there, that might be considered a business win in the coldest terms, but holy shit, what a terrible outcome for human care—even more soulless than our current health care machinery.
What if, instead, we change the goal to better care, lower health costs, and more employment? In that case, AI might still aid in intake, synthesize symptoms and test results, and provide a summary for medical review—so that medical staff don’t have to do as much rote data entry and summation.
But THEN the doctor or physician’s assistant comes in. Because the machines have already done the initial medical analysis, the caregiver’s role is to deliver the message in a way that is caring and warm. Their time can be spent on letting patients tell their stories. Instead of a rushed five minutes with a doctor, the patient will get time to feel heard, ask questions, get info, be reassured.
And perhaps that caregiver doesn’t need as much education as doctors today, because they are supported by knowledgeable systems. That in turn makes health care less expensive for the patient. It also means we could afford more caregivers, for more jobs. Instead of using AI to reduce human contact, in other words, we can use the technology to create the circumstances for better, more humane connection in the times and contexts when people can be so much more effective than machines. At the same time, we can also reduce costs and increase employment.
But that won’t happen on its own. We first have to talk about it. We have to decide what’s important and what our vision should be. Here’s how Wagstaff puts it:
What’s missing is a discussion about what we want our technology to do for us. This is not a discussion about AI; it’s a discussion about where we want our world to go. This seems obvious, but nearly always the discussion doesn’t happen — partly because of our technology fetish, but also because entrenched interests will not be honest about what might happen. We’ve never had a proper debate about the pernicious effects of Western-built social media, but our politicians are happy to wave angry fingers at China over TikTok. …
AI is not a distant concept. It is fundamentally changing our lives at a clip we’ve never experienced. To allow those developing AI to lead the debate about its future is an error we may not get a chance to correct.
Nobody Wants To Work with Our Best Engineer
∞ Apr 20, 2024If Jon was a great engineer, why was he so hard to work with? Isn’t his job to get things right?
No. The job of an engineer is to get things done. And getting anything done past a certain point requires working well with others.
If you are right but nobody wants to work with you, what net value are you bringing to the team? …
Kindness is a valuable trait. Practice it.
Designing, building, and delivering digital experiences is hard, but it turns out the biggest challenges are almost always human, not technical. Are you making things better or worse? How can you improve collaboration and understanding to make your team more successful in realizing shared vision?