AI Guesses Whether You're Gay or Straight from a Photo
∞ Sep 9, 2017Well this seems ominous. The Guardian reports:
Artificial intelligence can accurately guess whether people are gay or straight based on photos of their faces, according to new research that suggests machines can have significantly better “gaydar” than humans.
The study from Stanford University – which found that a computer algorithm could correctly distinguish between gay and straight men 81% of the time, and 74% for women – has raised questions about the biological origins of sexual orientation, the ethics of facial-detection technology, and the potential for this kind of software to violate people’s privacy or be abused for anti-LGBT purposes.
The Pop-Up Employer: Build a Team, Do the Job, Say Goodbye
∞ Aug 2, 2017Big Medium is what my friend and collaborator Dan Mall calls a design collaborative. Dan runs his studio Superfriendly the same way I run Big Medium: rather than carry a full-time staff, we both spin up bespoke teams from a tight-knit network of well-known domain experts. Those teams are carefully chosen to meet the specific demands of each project. It’s a very human, very personal way to source project teams.
And so I was both intrigued and skeptical to read about an automated system designed to do just that at a far larger scale. Noam Scheiber reporting for The New York Times:
True Story was a case study in what two Stanford professors call “flash organizations” — ephemeral setups to execute a single, complex project in ways traditionally associated with corporations, nonprofit groups or governments. […]
And, in fact, intermediaries are already springing up across industries like software and pharmaceuticals to assemble such organizations. They rely heavily on data and algorithms to determine which workers are best suited to one another, and also on decidedly lower-tech innovations, like middle management. […]
“One of our animating goals for the project was, would it be possible for someone to summon an entire organization for something you wanted to do with just a click?” Mr. Bernstein said.
The fascinating question here is how systems might develop algorithmic proxies for the measures of trust, experience, and quality that weave the fabric of our professional networks. But even more intriguing: how might such models help to connect underrepresented groups with work they might otherwise never have access to? For that matter, how might those models introduce me to designers outside my circle who might introduce more diverse perspectives into my own work?
The BBQ and the Errant Butler
∞ Aug 2, 2017Marek Pawlowski shares a tale of a dinner party taken hostage by a boorish Alexa hell-bent on selling the guests music.
Amid the flashy marketing campaigns and rapid technological advances surrounding virtual assistants like Alexa, Cortana and Siri, few end users seem willing to question how the motivation of their creators is likely to affect the overall experience. Amazon has done much to make Alexa smart, cheap and useful. However, it has done so in service of an over-arching purpose: retailing. Of course, Google, Microsoft and Apple have ulterior motives for their own assistants, but it should come as no surprise that Alexa is easily sidetracked by her desire to sell you things.
Toy Story Lessons for the Internet of Things
∞ Aug 2, 2017Dan Gärdenfors ponders how to handle “leadership conflicts” in IoT devices:
In future smart homes, many interactions will be complex and involve combinations of different devices. People will need to know not only what goes on but also why. For example, when smart lights, blinds and indoor climate systems adjust automatically, home owners should be able to know what triggered it. Was it weather forecast data or the behaviour of people at home that made the thermostat lower the temperature? Which device made the decision and told the others to react? Especially when things donât end up the way we want them to, smart objects need to communicate more, not less.
As we introduce more sensors, services, and smart gadgets into our life, some of them will inevitably collide. Which one “wins”? And how do we as users see the winner (or even understand that there was a conflict in the first place)?
UX design gets complicated when you introduce multiple triggers from multiple opinionated systems. And of course all those opinionated systems should bow to the most important opinion of all: the user’s. But even that is complicated in a smart-home environment where there are multiple users who have changing needs, desires, and contexts throughout the day. Fun!
Politics Are a Design Constraint
∞ Aug 2, 2017Designers, if you believe that politics don’t belong at work, guess what: your work is itself political. Software channels behavior, and that means that it’s freighted with values.
Ask yourself: as a designer, what are the behaviors I’m shaping, for what audience, to what end, and for whose benefit? Those questions point up the fact that software is ideological. The least you can do is own that fact and make sure that your software’s politics line up with your own. John Warren Hanawalt explains why:
Designers have a professional responsibility to consider what impact their work has—whether the project is explicitly “political” or not. Design can empower or disenfranchise people through the layout of ballots or UX of social network privacy settings.
Whose voices are amplified or excluded by the platforms we build, who profits from or is exploited by the service apps we code, whether we have created space for self-expression or avenues for abuse: these are all political design considerations because they decide who is represented, who can participate and at what cost, and who has power. […]
If you’re a socially conscious designer, you don’t need to quit your job; you need to do it. That means designing solutions that benefit people without marginalizing or harming others. When your boss or client asks you to do something that might do harm, you have to say no. And if you see unethical behavior happening in other areas of your company, fight for something better. If you find a problem, you have a problem. Good thing solving problems is your job.
AI Firstâwith UX
∞ Aug 2, 2017When mobile exploded a decade ago, many of us wrestled with designing for the new context of freshly portable interfaces. In fact, we often became blinded by that context, assuming that mobile interfaces should be optimized strictly for on-the-go users: we overdialed on location-based interactions, short attention spans, micro-tasks. The “lite” mobile version ruled.
It turned out that the physical contexts of mobile gadgetsâdevice and environmentâwere largely red herrings. The notion of a single “mobile context” was a myth that distracted from the more meaningful range of “softer” contexts these devices introduced by unchaining us from the desktop. The truth was that we now had to design for a huge swath of temporal, behavioral, emotional, and social contexts. When digital interfaces can penetrate any moment of our lives, the designer can no longer assume any single context in which it will be used.
This already challenging contextual landscape is even more complicated for predictive AI assistants that constantly run in the background looking for moments to provide just-in-time info. How much do they need to know about current context to judge the right moment to interrupt with (hopefully) useful information?
In an essay for O’Reilly, Mike Loukides explores that question, concluding that it’s less a concern of algorithm design than of UX design:
What’s the experience I want in being âassistedâ How is that experience designed? A design that requires me to expend more effort to take advantage of the assistant’s capabilities is a step backward.
The design problem becomes more complex when we think about how assistance is delivered. Norvig’s "reminders" are frequently delivered in the form of asynchronous notifications. That’s a problem: with many applications running on every device, users are subjected to a constant cacophony of notifications. Will AI be smart enough to know what notifications are actually wanted, and which are just annoyances? A reminder to buy milk? That’s one thing. But on any day, there are probably a dozen or so things I need, or could possibly use, if I have time to go to the store. You and I probably don’t want reminders about all of them. And when do we want these reminders? When we’re driving by a supermarket, on the way to the aforementioned doctor’s appointment? Or would it just order it from Amazon? If so, does it need your permission? Those are all UX questions, not AI questions.
We’ve made lots of fast progress in just the last few yearsâmonths, evenâin crafting remarkably accurate algorithms. We’re still getting started, though, in crafting the experiences we wrap around them. There’s lots of work to be done right now by designers, including UX research at unprecedented scale, to understand how to put machine learning to use as design material. I have ideas and design principles about how to get started. In the meantime, I really like the way Mike frames the problem:
In a future where humans and computers are increasingly in the loop together, understanding context is essential. But the context problem isn’t solved by more AI. The context is the user experience. What we really need to understand, and what we’ve been learning all too slowly for the past 30 years, is that technology is the easy part.
Making Software with Casual Intelligence
∞ Aug 2, 2017The most broadly impactful technologies tend to be the ones that become mundane—cheap, expected, part of the fabric of everyday life. We absorb them into our lives, their presence assumed, their costs negligible. Electricity, phones, televisions, internet, refrigeration, remote controls, power windows—once-remarkable technologies that now quietly improve our lives.
That’s why the aspects of machine learning that excite me most right now are the small and mundane interventions that designers and developers can deploy today in everyday projects. As I wrote in Design in the Era of the Algorithm, there are so many excellent (and free!) machine-learning APIs just waiting to be integrated into our digital products. Machine learning is the new design material, and it’s ready today, even for the most modest product features.
All of this reminds me of an essay my friend Evan Prodromou wrote last year about making software with casual intelligence. It’s a wonderful call to action for designers and developers to start integrating machine learning into everyday design projects.
Programmers in the next decade are going to make huge strides in applying artificial intelligence techniques to software development. But those advances aren’t all going to be in moonshot projects like self-driving cars and voice-operated services. They’re going to be billions of incremental intelligent updates to our interfaces and back-end systems.
I call this _casual intelligence_ — making everything we do a little smarter, and making all of our software that much easier and more useful. It’s casual because it makes the user’s experience less stressful, calmer, more leisurely. It’s also casual because the developer or designer doesn’t think twice about using AI techniques. Intelligence becomes part of the practice of software creation.
Evan touches on one of the most intriguing implications of designing data-driven interfaces. When machines generate both content and interaction, they will often create experiences that designers didn’t imagine (both for better and for worse). The designer’s role may evolve into one of corralling the experience in broad directions, rather than down narrow paths. (See conversational interfaces and open-ended, Alexa/Siri-style interactions, for example.)
Designers need to stop thinking in terms of either-or interfaces — either we do it this way, or we do it that way. Casual intelligence lets interfaces become _and-also_ — different users have different experiences. Some users will have experiences never dreamed of in your wireframes — and those may be the best ones of all.
In the AI Age, “Being Smart” Will Mean Something Completely Different
∞ Aug 2, 2017As machines become better than people at so many things, the natural question is what’s left for humans—and indeed what makes us human in the first place? Or more practically: what is the future of work for humans if machines are smarter than us in so many ways? Writing for Harvard Business Review, Ed Hess suggests that the answer is in shifting the meaning of human smarts away from information recall, pattern-matching, fast learning—and even accuracy.
What is needed is a new definition of being smart, one that promotes higher levels of human thinking and emotional engagement. The new smart will be determined not by what or how you know but by the quality of your thinking, listening, relating, collaborating, and learning. Quantity is replaced by quality. And that shift will enable us to focus on the hard work of taking our cognitive and emotional skills to a much higher level.
We will spend more time training to be open-minded and learning to update our beliefs in response to new data. We will practice adjusting after our mistakes, and we will invest more in the skills traditionally associated with emotional intelligence. The new smart will be about trying to overcome the two big inhibitors of critical thinking and team collaboration: our ego and our fears. Doing so will make it easier to perceive reality as it is, rather than as we wish it to be. In short, we will embrace humility. That is how we humans will add value in a world of smart technology.
In a Few Years, No Investors Are Going To Be Looking for AI Startups
∞ Jul 9, 2017Frank Chen of Andreessen Horowitz suggests that while machine learning and AI are today’s new hotness, they’re bound to be the humdrum norm in just a few short years. Products that don’t have it baked in will seem oddly quaint:
Not having state-of-the-art AI techniques powering their software would be like not having a relational database in their tech stack in 1980 or not having a rich Windows client in 1987 or not having a Web-based front end in 1995 or not being cloud native in 2004 or not having a mobile app in 2009. In other words, in a small handful of years, software without AI will be unthinkable.
So ambitious founders will need to invest some other way to differentiate themselves from the crowd — and investors will be looking for other ways to decide whether to fund a startup. And investors will stop looking for AI-powered startups in exactly the same way they don’t look for database-inside or cloud-native or mobile-first startups anymore. All those things are just assumed.
As Chen says, this feels like mobile just a few years ago. Just as mobile was the oxygen feeding emerging interactions and capabilities, machine learning is doing the same now. All the new interactions, all the new digital superpowers, they’re all being fueled by machine learning and algorithms.
In 2012, I wrote a chapter for The Mobile Book, for which Jeremy Keith wrote a prescient foreword. “This book is an artefact of its time,” he wrote. “There will come a time when this book will no longer be necessary, when designing and developing for mobile will simply be part and parcel of every Web worker’s lot.”
Yep, five years later, mobile is an assumed part of the job. If you were writing a “Machine Learning Book” today, you could borrow the same observation for the foreword. It’s time to get your game on now, since this will be an assumed capability in short order.
If you’re a designer wondering how you fit into all of this, I have some ideas: Design in the era of the algorithm.
Airlines Redesigning Uniforms Find Out How Complicated It Is
∞ Jul 9, 2017I’m a fan of the commitment, iteration and heavy testing that goes into the design of airline uniforms. Martha C. White reports for The New York Times that the process can take 2–3 years from start to finish.
Uniforms also have to reflect the realities of life on the road, with fabric blends that resist stains and wrinkles and can be laundered, if necessary, in a hotel sink. They also need to keep the wearers comfortable, whether their plane touches down in the summer in Maui or in the winter in Minneapolis.
Before giving the new uniforms to employees, the airlines conduct wear tests. The roughly 500 employees in American’s test reported back on details that needed to be changed. For example, Mr. Byrnes said, an initial dress prototype included a back zipper, but flight attendants found it challenging to reach. So the zipper was scuttled in favor of buttons on the front.
For its 1,000-employee wear test, Delta solicited feedback via surveys, focus groups, an internal Facebook page and job shadowing, in which members of the design team traveled with flight crews to get a firsthand view of the demands of the job.
“We had about 160-plus changes to the uniform design” as a result of those efforts, Mr. Dimbiloglu said.
The depth of the process makes sense because these uniforms define not only the company brand, but also impact the working life of thousands of people. Come to think of it, that’s true of pretty much any enterprise software, too. If you’re the designer of such things, are you bringing the same commitment to research, testing, and refinement to your software projects?
Uncovering Voice UI Design Patterns
∞ Jul 9, 2017The folks at Cooper are learning by doing as they experiment with building their own voice apps for Alexa and other platforms. As they’ve begun to encounter recurring problems, they’re taking note of the design patterns that solve them.
We’re trying to capture some of these patterns as we work on voice UI design for young platforms like Alexa. We identified five patterns and what they’re best suited for here.
This is how the industry finds its way to best practices: experimenting, sharing solutions, and finally, putting good names to those solutions. Cooper is off to a good start with these design patterns:
- A la carte menu
- Secret menu
- Confident command
- Call and response
- Educated guess
Surface Deep
∞ Jul 9, 2017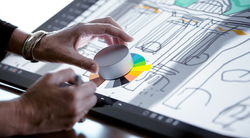
Ross Ufberg takes a deep dive into the design process behind Microsoft’s hyper-swiveling Surface Studio, a high-concept device that turns the desktop PC into a drafting table. A key ingredient to the project’s breakthrough success seems to be the highly collaborative, cross-disciplinary team that they corralled into Microsoft’s Building 87 to invent the thing:
Under one roof, Microsoft has united a team of designers, engineers, and prototypers, and invested heavily in infrastructure and equipment, so that Building 87 can be a self-contained hub, complete with manufacturing capabilities that usually would be located offsite or outsourced. Having these capabilities close at hand drastically cuts down on dead time, so that, in some cases, mere hours after a designer sends a concept down the hall to the prototypers, they can figure out a way to embody that concept, and print it in 3D or manufacture it on the spot. The model-making team can then hand that iteration off to the mechanical engineers, who assess the viability of the concept and figure out ways to improve it. It’s sort of like one endless feedback loop, with designers conceiving, prototypers creating, engineers correcting, and back again to the designers.
This is exactly the spirit of Big Medium’s own (far smaller) design teams. We have constant collaboration and feedback among product designers, visual designers, front-end designers, and developers. It’s not a linear process, but a constant conversation that blends experiments, false starts, grand leaps, successes, and gradual improvements. This turns out to be both faster and more creative.
In workshops and design engagements, we coach client organizations how to adopt this collaborative, iterative design process. Designers and developers often tell us at first that it feels unfamiliar, even uncomfortable, to collaborate across the entire design process—and especially when ideas are being formed. It’s natural to shy away from sharing before something is fully thought out. But that’s exactly where the most productive cross-disciplinary experiments happen.
One of Surface Studio’s signature design innovations is the hinge that lets it shift instantly from upright desktop monitor to a sloped, dial-and-stylus drafting board. In Ufberg’s telling, it wouldn’t have happened at all without a culture of cross-disciplinary experimentation.
“It is way easier to try something than tell somebody it can’t be done.”
When the idea of a hinge was first tossed out in a brainstorming meeting, [mechanical engineering director Andrew] Hill tells me, “there were ten different people who said that it doesn’t make any sense, it would be too complicated to make it work. But then a couple weeks later, we got a prototype out of our model shop where you could see the mechanism starting to come together, and the people who were saying it couldn’t be done started to come over and be like ‘Huh, maybe we could do something like this.’” I ask him if he was one of those doubters.
“One of the things that I found out about myself is it is way easier to try something than tell somebody it can’t be done,” he confesses. “There’s magic in the suspension of disbelief. If you just do stuff that you know you’re going to be able to do, you know where you’re going to go. If you try something that you’re not quite sure is going work, at least you’re exposed to new problems and you get smarter in that way, and in the good cases, you move the whole thing forward.” In its first iteration, the hinge was just a piece of cardboard glued crudely to a kickstand. But then, the feedback loop kicked into place.
The bottom line: When in doubt… don’t doubt. Build a quick prototype—the most low-fi thing you can create to test the concept—and then share it with people from other disciplines. It’s how you manage risk in an inherently risky exploration into the new.
That’s good advice not only for industrial design, but for pretty much anytime you’re really trying to make something new and better. And isn’t that all of us?
Need help transforming your organization’s design process for faster and more creative results? That’s what we do! Get in touch for a workshop, executive session, or design engagement.
Oil City High School 2017 Commencement Speech
∞ Jul 9, 2017Returning to scenes of youth is always complicated business, the stuff that makes high school reunions emotionally fraught. How have I changed, how haven’t I, and how do I express those things when I come home? I know Brad Frost was sweating these topics as he toiled over his commencement speech at the high school where he graduated 14 years ago.
Months before he gave the talk, he told me he was already nervous about it. Turns out he didn’t need to worry. In fact, the “what has/hasn’t changed” anxiety turned out to be central to his wonderful speech. I especially loved this message:
The things you will be doing in 14 years’ time will no doubt be different than the things you’re doing at this phase in your life. A recent study by the Department of Labor showed that 65% of students going through the education system today will work in jobs that haven’t been invented yet. Think about that. That means that the majority of today’s students — probably including the majority of this graduating class — will end up working in jobs that don’t presently exist. Technology is advancing at a staggering rate, it’s disrupting industries, it’s inventing new ones, and it’s constantly changing the way we live and work.
When I was a kid, I didn’t say “Mom, Dad, I want to be a web designer when I grow up!” That wasn’t a thing. And yet that’s now how I spend most of my waking hours, and how I earn my living, and how I provide for my family.
Our daughter Nika is about to start her final year of high school, and she sometimes worries that she doesn’t yet have enough vision for what she’ll become in her life and career—that she’s behind. But she knows what she loves, and she has so many talents, so we try to reassure her that knowing her skills, values, and personality is far more important than knowing a vocation. Vocations shift far more quickly than the rest.
When I graduated from high school (30 years ago next year!), the web hadn’t been invented, mainstream email was years away, and phones were cabled to the wall. But even then, I had a passion for both storytelling and systems—and those have been the guiding threads of a career spanning many kinds of jobs, culminating (for now at least!) in work shaping experiences of and for connected devices.
The great thing about returning to scenes of youth is that sometimes—like Brad—you get to talk to the kids coming up behind you. You can share the advice you’d offer your younger self. Nice work, Brad.
So We Redid Our Charts…
∞ Jun 28, 2017Video analytics provider Mux overhauled their data visualizations and shared the process. The thinking and results make for a worthwhile read, but I was especially taken with the project’s framing questions:
When we wanted to revisit our charts, we looked at them from both of these perspectives and asked ourselves: Are we being truthful to our calculations? And, are we presenting the data in a beautiful and sensible manner?
I’m more convinced than ever: the presentation of data is just as important as the underlying algorithm.
Google Will No Longer Scan Gmail for Ad Targeting
∞ Jun 28, 2017In a bit of unexpected good news, Google announced last week that it will stop reading Gmail messages for the purpose of showings ads tailored to the content.
The New York Times reminds, however, that Google will still continue to read your messages for other purposes:
It will continue to scan Gmail to screen for potential spam or phishing attacks as well as offering suggestions for automated replies to email.
So the bots will continue to comb your email missives, but at least they’re seemingly doing this in the service of features that serve the user (instead of advertisers).
As always, the tricky trade-off of machine-learning services is that they only know what we feed them. But what are they eating? It’s us. How much are we willing to reveal, and in exchange for what services?
A Working Pattern Library
∞ Jun 28, 2017The charming and masterful Ethan Marcotte emphasizes the importance of crafting design systems to fit the way an organization works and behaves:
To put a finer point on it, a pattern library’s value to an organization is tied directly to how much—and how easily—it’s used. That’s why I usually start by talking with clients about the intended audiences for their pattern library. Doing so helps us better understand who’ll access the pattern library, why they’ll do so, and how we can design those points of contact.
While a basic pattern library might consist simply of a collection of components, an essential pattern library is presented in a way that makes it not just the “right” thing to use but the easiest. For what it’s worth, this is really hard to do well; as we’ve found in our own projects, there’s some seriously demanding UX/research work behind tailoring a design system to the people who use it.
On Design Systems: Dan Mall of Superfriendly
∞ Jun 28, 2017UXpin interviewed my friend and collaborator Dan Mall to tap his giant brain for insights about building design systems. The 47:15 interview is full of gems, and you should watch or read the whole thing. Meanwhile, here are a few highlights:
I don’t think that a design system should remove design from your organization. A lot of clients think like, “Oh, if we had a design system, we don’t need to design anymore, right? ‘Cause all the decisions would be made.” And I think that I’ve never seen that actually happen. Design systems should just help you design better. So you’re still gonna have to go through the process of design, but a design system should be a good tool in your arsenal for you to be able to design better. And eliminate some of the useless decisions that you might have to make otherwise.
I love this. Design systems are at their best when they simply gather the best practices of the organization—the settled solutions. The most effective design systems are simply containers of institutional knowledge: “this is what good design looks like in our company.” Instead of building (and rebuilding and rebuilding) the same pattern over and over again, designers and developers Instead of designing a card pattern for the 15th time, it’s already done. Product teams can put their time and smarts into solving new problems. Put another way: the most exciting design systems are boring.
Dan also talks about the importance of making the system fit the workflow of the people who use it. In our projects together, we’ve found that deep user research is required to get it right:
I’ve worked with organizations that the design systems are for purely developers. That’s it. It’s not for designers. And then in other organizations, a design system has to work equally well for designers and developers. And so just those two examples, those two design systems have to be drastically different from each other ’cause they need to support different use cases and different people.
If that kind of user-centered research sounds like product work, that’s because it is:
It is a product and you have to treat it like a product. It grows like a product, it gets used like a product. And for people to think like, “Yeah we’ll just create it once and then it’ll sit there.” They’re not realizing the value of it and they may not actually get value from it if they’re not treating it like a thing that also needs to grow in time and adapt over time. … The reason that the design system is so hard to get off the ground is because it requires organizational change. It requires people to have different mindsets about how they’re going to work.
The Future of AI Needs To Have More People in It
∞ Jun 28, 2017If smart machines and AI agents are meant to support human goals, how might we help them better understand human needs and behaviors? UC Berkeley professor and AI scientist Anca Dragan suggests we need a more human-centered approach in our algorithms:
Though we’re building these agents to help and support humans, we haven’t been very good at telling these agents how humans actually factor in. We make them treat people like any other part of the world. For instance, autonomous cars treat pedestrians, human-driven vehicles, rolling balls, and plastic bags blowing down the street as moving obstacles to be avoided. But people are not just a regular part of the world. They are people! And as people (unlike balls or plastic bags), they act according to decisions that they make. AI agents need to explicitly understand and account for these decisions in order for them to actually do well. […]
How do we tell a robot what it should strive to achieve? As researchers, we assume we’ll just be able to write a suitable reward function for a given problem. This leads to unexpected side effects, though, as the agent gets better at optimizing for the reward function, especially if the reward function doesn’t fully account for the needs of the people the robot is helping. What we really want is for these agents to optimize for whatever is best for people. To do this, we can’t have a single AI researcher designate a reward function ahead of time and take that for granted. Instead, the agent needs to work interactively with people to figure out what the right reward function is. […]
Supporting people is not an after-fix for AI, it’s the goal. To do this well, I believe this next generation should be more diverse than the current one. I actually wonder to what extent it was the lack of diversity in mindsets and backgrounds that got us on a non-human-centered track for AI in the first place.