The More You Use Facebook, the Worse You Feel
∞ Apr 11, 2017In Harvard Business Review, Holly B. Shakya and Nicholas A. Christakis report the results of their rigorous study of Facebook use:
Overall, our results showed that, while real-world social networks were positively associated with overall well-being, the use of Facebook was negatively associated with overall well-being. These results were particularly strong for mental health; most measures of Facebook use in one year predicted a decrease in mental health in a later year. We found consistently that both liking others’ content and clicking links significantly predicted a subsequent reduction in self-reported physical health, mental health, and life satisfaction.
Our models included measures of real-world networks and adjusted for baseline Facebook use. When we accounted for a person’s level of initial well-being, initial real-world networks, and initial level of Facebook use, increased use of Facebook was still associated with a likelihood of diminished future well-being. This provides some evidence that the association between Facebook use and compromised well-being is a dynamic process.
Be careful out there.
Web Performance Optimization Stats
∞ Apr 11, 2017WPO Stats is a super-useful collection of stats from Tammy Everts and Tim Kadlec to demonstrate the business value of faster websites. If you need support for making the business case for your performance project, here’s your go-to library.
A sampling:
BBC has seen that they lose an additional 10% of users for every additional second it takes for their site to load. [source]
…
AliExpress reduced load time by 36% and saw a 10.5% increase in orders and a 27% increase in conversion for new customers. [source]
…
For every 100ms decrease in homepage load speed, Mobify’s customer base saw a 1.11% lift in session based conversion, amounting to an average annual revenue increase of $376,789. [source]
Google Launches New Effort To Flag Upsetting or Offensive Content in Search
∞ Apr 10, 2017I missed this a few weeks back. At Search Engine Land, Danny Sullivan reported that Google is empowering its 10,000 human reviewers to start flagging offensive content, an effort to get a handle on hate speech in search results. The gambit: with a little human help from these “quality raters,” the algorithm can learn to identify what I call hostile information zones.
Sullivan writes:
The results that quality raters flag is used as âtraining dataâ for Googleâs human coders who write search algorithms, as well as for its machine learning systems. Basically, content of this nature is used to help Google figure out how to automatically identify upsetting or offensive content in general.â¦
Google told Search Engine Land that has already been testing these new guidelines with a subset of its quality raters and used that data as part of a ranking change back in December. That was aimed at reducing offensive content that was appearing for searches such as âdid the Holocaust happen.â
The results for that particular search have certainly improved. In part, the ranking change helped. In part, all the new content that appeared in response to outrage over those search results had an impact.
âWe will see how some of this works out. Iâll be honest. Weâre learning as we go,â [Google engineer Paul Haahr] said.
The Most Successful Interface Design of All Time
∞ Apr 10, 2017In ~20 years, Google Search lost some cruft and gained speech recognition, but the fundamental design of the entry page is virtually identical.
“Fast, easy, and useful beats all,” Erika writes.
Amen. What’s the job of the page? How can you focus exclusively on that and cut out the extraneous?
Federated Learning: Collaborative Machine Learning without Centralized Training Data
∞ Apr 9, 2017Google researchers Brendan McMahan and Daniel Ramage report that Google has begun offloading some of its machine learning to mobile devices to keep private data… private. Instead of pulling all your personal info into a central system to train its algorithms, Google has developed the chops to let your phone do that data analysis. They call it federated learning:
Federated Learning allows for smarter models, lower latency, and less power consumption, all while ensuring privacy. And this approach has another immediate benefit: in addition to providing an update to the shared model, the improved model on your phone can also be used immediately, powering experiences personalized by the way you use your phone.
We’re currently testing Federated Learning in Gboard on Android, the Google Keyboard. When Gboard shows a suggested query, your phone locally stores information about the current context and whether you clicked the suggestion. Federated Learning processes that history on-device to suggest improvements to the next iteration of Gboard’s query suggestion model.
Old way: beam everything you do on your Google keyboard (!!) back to the mothership. New way: keep it all local, and beam back only an encrypted summary of relevant learnings. “Your device downloads the current model, improves it by learning from data on your phone, and then summarizes the changes as a small focused update.” To do this, Google has smartphones running a minature version of TensorFlow, the open-source software library for machine learning .
One knock against predictive interfaces is how much you have to give up about yourself to get the benefits. If this new model works as promised, new systems may be just as helpful, without the central service absorbing your nitty-gritty details to learn how.
The Full Stack Design System
∞ Apr 9, 2017Emmet Connolly shared some wonderful thoughts about pattern libraries—and how they’re only one part of a full design system:
But there are a few problems with pattern libraries. Yes, they allow you to keep all of the smallest elements consistent. But they don’t have an opinion about how they should be put together. They don’t know anything about your product or the concepts behind it.
To return to our Lego analogy, simply having a limited pattern library of bricks to choose from doesn’t preclude me from building some really crazy shit.
Now think about those branded Lego kits you can buy. Each piece is much more opinionated. It knows what it’s going to get used for. There are still generic pieces involved, but when you put them together in a certain way they form something specific, like the leg of an AT-AT Walker. This is a design system.
I love it. Design systems are more than a kit of parts. The best design systems have a strong point of view—a gravitational force that coerces disparate components into patterns and ultimately into a coherent whole. The design system brings order to the pattern library and what would otherwise appear to be a chaotic jumble of components.
Another metaphor: if components are words, then patterns are sentences, and the design system is the full story.
If this nested arrangement echoes Brad Frost’s Atomic Design methodology, that’s by design. Atomic Design champions design elements built from a common set of lesser design elements. In Atomic Design, UI “atoms” assemble “molecules” which assemble “organisms” which assemble templates which assemble pages.
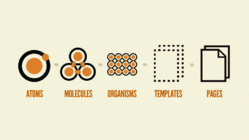
But there’s a common misunderstanding about Atomic Design which Connolly in turn suggests is a limitation:
Atomic Design will tell you to take some of your basic elements (label, input, button), stick them together, and call it a molecule. Then you can reuse that molecule again and again. Further, you can stick some molecules together to form a reusable organism.
The problem with every real-world example of a system like this that I’ve encountered is that they remain willfully unaware of the product being built.
Atomic Design does indeed promote reuse, assembling larger parts from smaller ones. However, many mistake this philosophy for linear process, that somehow Atomic Design demands that all design must first start by building its smallest pieces (e.g. “start with buttons and labels”) before proceeding to page- and site-level design. It’s an approach that would indeed be blind to the end-result project, placing design tactics ahead of design strategy. But that’s exactly opposite to how Brad himself approaches projects.
It’s never a linear path from small to large; it’s a constant roundtrip between the two scales.
Right from the start, when Brad was first developing his tools and methodologies in our designs of TechCrunch and Entertainment Weekly, our process constantly zoomed back and forth between page level and atomic level. It’s never a linear path from small to large; it’s a constant roundtrip between the two scales.
As Connolly writes, “Complex systems can be designed, but to do so you must first sketch the outline. Only then can you start filling in the detail.”
Well said, and I totally agree. Indeed, our Atomic Design projects always begin with the big-picture questions. What are the business goals for the project? What are the user needs? What’s the brand promise? When we get to individual pages, it’s about the user mindset when they arrive, and the jobs the page has to do for both user and company.
From there, we do sketching of the whole page, identifying the broad design patterns that the page needs to do its job. We start to imagine the components necessary to bring those patterns to life.
Only then do we start to work at the atomic level, building out those component atoms and molecules to construct the pattern organisms, and ultimately the page itself. As more high-level pages and components are designed, we zoom back down to revisit the atoms and molecules, making adjustments to make them more flexible and support a wider range of organisms and pages. The atoms and molecules might compose the design, but it’s the high-level design that creates the order, the overall system.
In the end, a pattern library emerges. Here’s the important bit: the design system is implicit in the process that led to the library’s construction, and it’s implicit in the design’s use of components. For a small team on a contained project, that implicit knowledge may well be enough, commonly shared in the heads of the designers who built it.
But implicit knowledge won’t do when you’re working at scale across many projects and many teams. The design system has to be documented. That’s where all the other artifacts of a fully articulated design system come in: design principles, style guide, voice and tone, UX guidelines, code repository, and so on.
I agree very much with Connolly that those pieces are required for the “full-stack design system.” My only caveat is to add that an Atomic Design process can get you there, too.
Atomic Design surfaces all of those aspects during the course of the design process. Responsible designers document them.
For more, see The Most Exciting Design Systems Are Boring.
Is your organization wrestling with inconsistent interfaces and duplicative design work? Big Medium helps big companies scale great design through design systems. Get in touch for a workshop, executive session, or design engagement.
Sleep Is the New Status Symbol
∞ Apr 9, 2017At The New York Times, Penelope Green reports that sleep is big business—and the tech industry is rushing in to tweak our natural rhythms, with mixed results:
Mr. Mercier sent me his Dreem headset, a weighty crown of rubber and wire that he warned would be a tad uncomfortable. The finished product, about $400, he said, will be much lighter and slimmer. But it wasn’t the heft of the thing that had me pulling it off each night. It skeeved me out that it was reading — and interfering with — my brain waves, a process I would rather not outsource.
I was just as wary of the Re-Timer goggles, $299, which make for a goofy/spooky selfie in a darkened room. My eye sockets glowed a deep fluorescent green, and terrified the cat.
The science and research confirm that there’s an epidemic of sleeplessness, which is costly in both health and productivity. Are tech gadgets the answer when tech gadgets are likely a big part of the problem? Our screens keep us awake; always-on information demands contribute to anxiety and stress; and social FOMO is constant.
As technologists, we often suggest that more technology is the solution to technology’s problems. In the case of sleep, perhaps a little less technology is what’s needed. Green quotes “sleep ambassador” Nancy Rothstein:
“Your Fitbit and your Apple Watch are not going to do it for you. We’ve lost the simplicity of sleep. All this writing, all these websites, all this stuff. I’m thinking, Just sleep. I want to say: ‘Shh. Make it dark, quiet and cool. Take a bath.’”
YouTube To Discontinue Video Annotations Because They Never Worked on Mobile
∞ Apr 9, 2017At The Verge, Nick Stat reports that YouTube is dropping its video annotations:
“Annotations Editor launched in 2008, before the world went mobile,” writes YouTube product manager Muli Salem in a blog post. “With 60 percent of YouTube’s watchtime now on mobile, why go through the work of creating annotations that won’t even reach the majority of your audience?”
If it doesn’t work on mobile, it doesn’t work, period.
See also: Your Traffic Went Mobile; Why Hasn’t Your Design Process?
Self-Driving Cars Are Coming, but Self-Driving Tractors Are Already Here
∞ Apr 8, 2017Kaleigh Rogers for Motherboard:
Self-driving tractors are becoming more common. A John Deere spokesperson told me the company currently has about 200,000 self-driving tractors on farms around the world, from the US to Germany. And they’re just one example of a major investment that the agriculture sector is making in artificial intelligence and the Internet of Things.
According to John Deere, between 60 and 70 percent of the crop acreage in North America today is farmed using GPS-driven tractors. (Source)
Farmers have been inhabiting the future for a long time—self-driving tractors have been on the go for 15 years. Industrial farms are big business; they feature wide-open spaces; and they operate on private property. All make of this makes farms ideal test beds for tech that includes autonomous vehicles, drones, artificial intelligence, and smart objects.
Lightform: The Magical Little Device That Transforms Whole Rooms Into Screens
∞ Apr 8, 2017Liz Stinson, in Wired, previews Lightform, a “projection-mapping” device that can read a room and project images (or interfaces) onto any surface, no matter how irregular. In a nutshell, it’s augmented/mixed reality projected directly onto the environment:
Lightformâs technology sets the stage for more complex and immersive forms of interaction. The company aims to develop high-resolution augmented reality projections that track objects and respond to human input in real time. Its ultimate goal: Make projected light so functional and ubiquitous that it replaces screens as we know them in daily life life. âReally what weâre doing is bringing computing out into the real world where we live,â Sodhi says.
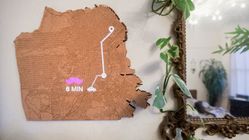
What I like about emerging technologies like this one is that the tech comes to you. Your surroundings simply become digital; no need to strap on a headset or peer through a screen.
Writing for MEX last week, Marek Pawlowski made a similar observation:
Virtual, augmented and mixed reality products like HoloLens and Daydream are often seen as being in the vanguard of this evolution, but the level of immersion required by these experiences is a somewhat misleading guide to the future.
The larger concept at play here is the notion that digital capabilities â through projection, augmentation or other more subtle forms of ingress â will become woven into the physical fabric of life. The dream of ubiquitous computing will not come in boxes, but rather will hover and shimmer in transient spaces around us.
“Woven into the physical fabric of life.” This is the exciting opportunity about the physical interface, whether embodied in IoT gadgets, projected UI, or augmented reality: it literally grafts onto the world around us, on our terms. It’s tech that promises to bend to our lives, rather than the reverse.
Notification System Design (99+)
∞ Apr 8, 2017Quora designer Henry Modisett shares perspectives on the unique challenges of designing effective, respectful notifications:
A notification is the product communicating with you while you are not using it. It is a naturally interruptive and invasive experience to various degrees. Because of that it is a very consequential system, meaning that every thing you send through it will have material impact on the user’s experience with your product.
I especially liked his caution about being responsible with notifications that are solely intended to goose engagement:
These are essentially advertisements. For example, any digest email. One common property of a notification that has an explicit engagement goal is that they don’t need to be sent, meaning that the user doesn’t necessarily have any expectation that they will come. This is what makes them powerful and dangerous. Most people have experienced some abuse of this by some app who has wielded this for some sort of short term gain. “Happy Valentine’s day, we love you, come check out our app today!”
It’s difficult to summarize this broad and thoughtful overview of the UX and psychology of notifications—read the whole thing—but I’ll call out a few nuggets:
- When the value of notifications are high enough, users will welcome incredibly high volume (e.g., text messages).
- Offering user preferences for notifications is hard: “When you have to design the settings for these things it all get exposed to the user how hedgy these decisions often are. You either end up with a small set of extremely vague settings, or you end up with a overwhelming display of different toggles in an attempt to give the user some sense of control.”
- Short-term engagement is misleading. More notifications always delivers more engagement, and so too many companies simplistically dial the notification machine way too high. It works until it doesn’t, users burn out.
- Notifications for today’s popular voice interfaces don’t really exist; all interactions are initiated by the user. This is both an opportunity and an unsolved problem.
Losing One’s Self in Selfie Moments
∞ Apr 8, 2017At MEX, Marek Pawlowski ponders the rapidly arrived ubiquity of the selfie. Now that selfies are so commonplace—peak selfie!—he asks a great question: what’s next?
What will be the next large-scale creative trend after selfies? The human desire to preserve themselves in a moment is timeless, but surely the smartphone snap is not the zenith of this desire for self-regard?
Alan Kay’s Answer to What Made Xerox PARC Special
∞ Apr 8, 2017At Quora, Alan Kay himself rather awesomely answers the question, what made Xerox PARC special?. Among many other things, Kay invented the modern graphical user interface (GUI) during his years at PARC, which is the birthplace of technologies including the laser printer, ethernet, and object-oriented programming.
Kay answers the question with a list of the principles that animated PARC’s early years. Among them:
- Visions not goals
- Problem finding, not just problem solving
- “It’s ‘baseball’ not ‘golf’ — batting .350 is very good in a high-aspiration, high-risk area. Not getting a hit is not failure but the overhead for getting hits.”
- Researchers should design and build their own tools
In an era when we tediously debate “should designers learn to code,” that last bullet might seem extreme. Kay would say designers should not only learn to code, they should learn to build hardware, too:
The idea was that if you are going to take on big important and new problems then you just have to develop the chops to pull off all needed tools, partly because of what “new” really means, and partly because trying to do workarounds of vendor stuff that is in the wrong paradigm will kill the research thinking.
To pull in a well-known Kay quote, “the best way to imagine the future is to build it.”
Fact Check Now Available in Google Search and News
∞ Apr 8, 2017Google announced that the search engine has begun giving special treatment to fact-checking websites in search results:
For the first time, when you conduct a search on Google that returns an authoritative result containing fact checks for one or more public claims, you will see that information clearly on the search results page. The snippet will display information on the claim, who made the claim, and the fact check of that particular claim.
So, for example, searching for “did millions of illegals vote” surfaces a fact-check article from politifact.com. The result for that article is captioned with a brief summary of the fact-check, finding the claim untrue that millions of non-citizens voted in the US presidential election:
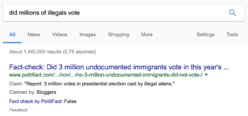
At a minimum, this seems like it will be a useful step in flagging misinformation, or facts in dispute. The presence of one or more fact-check results in a search at least hints that there’s bad information or cynical propaganda afoot.
More broadly, this may prove to be a foundation for doing more to identify hostile information zones—toxic topics that poison our civic discourse and confuse search engines. How might the presence of these results be highlighted even more to caution the reader to be alert or skeptical when exploring this topic?
The success of this depends on Google identifying genuinely trustworthy sites to get this call-out treatment. How all of this works: behind the scenes, Google is extracting structured data inserted into the page (specifically, markup using the ClaimReview schema). Any website can insert that fact-check markup, but Google says it’s giving the new treatment only to sites “algorithmically determined to be an authoritative source.”
Algorithms can be fooled and gamed, of course, which is part of our fake-news mess in the first place. The promising step of calling out fact-check information would be seriously undermined if search results started including white-supremacist sites “fact checking” the equality of races, for example.
As I wrote in Systems Smart Enough To Know When They’re Not Smart Enough, our answer machines need to work harder at signaling when their answers may be compromised—by either widespread misinformation or even outright manipulation. As I argued there, this is a challenge of design and presentation as much as machine learning. Google’s new tweak is a small but useful first step in improving presentation.
Worth noting: this fact-check approach may help address controversies and misunderstandings. However, it does not do much for other hostile information zones—the awful results and “answers” that Google delivers if you ask it if women or Jews are evil, for example. That kind of hate is not about “disputed facts.” Our answer machines will have to find other ways to highlight the toxicity of those topics and the illegitimacy of their sources. In the meantime, this new change may at least help take down more conventional misinformation.
See also: Facebook’s efforts to flag disputed news with third-party fact checkers and to offer tips for identifying fake news.
If your company is wrestling with how to present complex data with confidence and trust, that’s exactly the kind of ambitious problem that we like to solve at Big Medium. Get in touch.
There’s Nowhere to Hide on the Internet
∞ Apr 7, 2017Thomas Beller writes for the New Yorker about Internet Noise, a clever project that loads random pages in your browser to garbage-up your search history for advertisers or other snoopers. It’s digital camouflage for the precise moment that we’re all getting that creepy feeling we’re being watched.
“We live in a moment when our government has too little transparency and our own private lives have too much,” Beller writes. “Internet Noise is a cleaning appliance—even though it achieves cleanliness by creating an obscuring veil, a kind of digital squid ink. Internet Noise is scrubbing your traces online, removing the evidence of your real self.”
(As a secondary bonus, the service is also a reminder of just how odd and wonderful the internet is. Beller describes watching Internet Noise take his browser on a hypnotic journey through pages about anti-social birders, videos of lonely book readings, images of obscure paintings, and the details of Florida’s water aquifers.)
Alas, Internet Noise isn’t likely to be effective camouflage; it’s more an art-project protest statement. Its creator Dan Schultz tells Beller that the ad-surveillance apparatus is already too sophisticated to fall for simple tricks:
“Advertisers will know it’s a robot,” [Schultz] said. “This is a noise generator. We are talking about signal processing. Humans signal-process every second of every day. When I hear a sound, my brain is processing that sound. Noise does not affect the signal. It is around the signal. We might be annoyed by noise, but even if there is static on the radio we can still pick up the melody. We just might miss some of the subtle nuances. Same thing goes for your fingerprint online. The algorithms are able to tell.”
Tim Berners-Lee on Everything Wrong with the Web Today
∞ Apr 5, 2017Quartz sums up several interviews that Sir Tim Berners-Lee is giving this week. The web’s daddy is a little disappointed in how things are turning out. Berners-Lee specifically calls out three ways that the web isn’t living up to its ideals:
- Advertising’s pernicious effect on the news
- Social networks ignoring their responsibility to the truth
- Online privacy is a “human right” that’s being trampled
Mobile Web: Back To the Future
∞ Apr 5, 2017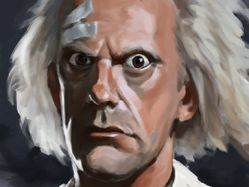
At mobiForge, Ronan Cremin surveys the last two decades of mobile web technology, from WAP to i-mode to responsive web design to Googleâs AMP platform. This long-view perspective reveals responsive web design to be a possible detour from an otherwise steady march of technologies that fork mobile into its own codebase, âthe mobile website.â
With AMP, Ronan suggests weâve kinda come full circle.
And thus here we are, WAP to the future, in a place that looks remarkably like where we started out in the 1990s, with many prominent websites now separately delivering made-for-mobile experience to mobile devices, be it AMP or Instant Articles. Given that there are already one billion AMP pages itâs probably safe to say that there is more mobile-specific content out there now than at the height of the m-dot era.
The ostensible driver behind every one of the mobile-specific platforms is performance. They all serve a separate set of code because mobile gadgets and their networks are slower than other devices.
And yet, thereâs little evidence that most publishers are interested in fast mobile experiences. Even as mobile has become far and away the biggest source of traffic, websites have grown heavier and heavier.
Performance is not the priority it should be, but responsive design is not the problem: ads and images are. The tech in platforms like AMP is largely about wrangling abusive JavaScript and handling smart caching, handling responsive images, and other responsible dev practices. This, of course, is the stuff that all of us should be doing, but that most of us arenât.
These platforms force the business decisions that publishers apparently wonât make on their own.
So it seems that performance is less the âdriverâ than the passenger when deciding to adopt these platforms. I totally agree with Ronan that the decision to adopt platforms like AMP and Facebook Instant Articles is largely about SEO, not performance:
Some will fret about splitting the web and say that we have regressed, but on the other hand we now have some really fast mobile sites that reach more devices and lower-end devices than ever before. Could we have done this without AMP or Instant Articles? Yes, of course. But we wouldnât haveâand despite swathes of evidence pointing to the importance of page speedâwe didnât. Instead we got relentlessly heavier and slower.
Which is betterâa web with adaptively-served formats or an abandoned web? Are AMP et al the ârightâ way to fix the problem? Probably not, but itâs working and no other solution is getting any traction. It seems that it takes SEO pressure from Google to instigate change.
So weâre getting performant sites that publishers didnât otherwise have the gumption to build themselves. Thatâs good, for sure. But at what cost?
Among several worrying things about AMP and the other new platform-hosted platforms is exactly that: theyâre platform hosted. It feels like a monopoly grab, strongly biased by the ad-driven business models of Google and Facebook. Publishers are giving up some agency and even their URLs, which is dangerous. (Iâm sure there are many folks at these platforms who are earnestly all about improving performance and user experience, but once all this content is absorbed into big platforms, Iâm not convinced that good things will follow.)
But also, yeah, Iâm one of the people Ronan mentions who âfret about splitting the web.â Iâd hate to see us adopt the retrograde idea that the mobile web experience should be less than the ârealâ web experience on the desktop. If anything, traffic tells us that the mobile experience is now the real one. Better, however, to create most experiences (and certainly publishing/media sites) as device agnostic. Thatâs a core principle of the web: it doesnât care what device you bring to it. Everyone is welcome.
Here we go again
Separate website vs single responsive design is an old argument, and I think the ârightâ thing to do is still what I suggested back when I wrote this in 2011 in Responsive Web Design or Separate Mobile Site? Eh. It Depends. Default to a single responsive site unless you have such unique opportunities or constraints that the mobile site is effectively a different application:
The bottom line is whether youâre really talking about the same website in the first place. Depending on what youâre building, a mobile website could be an entirely different animal than its desktop counterpart, addressing entirely different needs. The simple thing to remember is that layoutâ content. Great designs require considerable attention to both. Responsive web design cannot dictate content strategy, nor vice versa.
The case for making a separate websiteâfor any platformâis when you have an opportunity to make something that is more than and qualitatively differently from what you might make for other platforms. Thatâs usually a rare case; in most cases, a healthy dollop of progressive enhancement does the trick. (Progressive enhancement is not always easy to do, as Ronan points out, but then again, thatâs why weâre all paid the big bucks: to shoulder hard work on behalf of the best possible product.)
The troubling thing, though, is that none of these elements seem to be key factors for publishers in deciding whether to adopt AMP for mobile devices. Itâs not about user stories, device capabilities, developer effort, or building the right product. Itâs about SEO.
Yet again, ad-driven business models warp decisions to the detriment of the underlying product.
In that way, at least, this isnât a âback to the futureâ story. All of the past mobile-platform efforts were at least developed and adopted with the goal of providing the best possible experience to mobile users. With platforms like AMP, that goal seems to be secondary.
No matter what platform you choose, letâs at least carry forward the learnings and principles weâve taken from the last few years or responsive design. Ronan rounds em up:
- Donât limit the choices available to mobile devices.
- Responsiveness is a good thing.
- You canât assume context from device.
- Device-specific URLs are a bad thing.
- Smartphones and mobile networks arenât as fast as you think.
Need help sorting out your mobile experience? We can help! Big Medium specializes in multiplatform design. Get in touch for an executive session, workshop, or design engagement.
The Web Looks Like Sh*t
∞ Apr 5, 2017At The Outline, Hanson O’Haver bemoans the abusive state of web design: popovers, auto-play videos, ads that crowd out content, swarms of cynical dark patterns:
You’ll notice that these problems with web design are mostly found on websites that depend on traffic and advertising. You don’t see these issues on Amazon, Google, or Facebook, which are constantly — if not always successfully — trying to redesign to make their sites easier and more pleasant to use. These companies are focused on maximizing eyeballs, which means any aesthetic improvements that don’t directly boost traffic are not a priority.
The piece gives a shout to our work at About.com (now Dotdash), where we designed several verticals and purposely “de-monetized” them—industryspeak for pulling out all those hideous ads. The result was a better user experience for readers (duh), but also a better experience for advertisers. When we pulled out the Outbrain/Taboola links, the crummy text links, and the zillion low-grade banner ads, the remaining ads got stronger placements, crafted to fit the design organically.
And get this: revenue is up because the sites have fewer ads. “The sales teams’ phones are ringing off the hook,” About CEO Neil Vogel tells us. Advertisers want more of this. They want more of less. Just like the rest of us. Everyone wins.
Too many sites let advertising corrupt their product, poisoning not only user experience but also the editorial (clickbait). Our recent work tells us it doesn’t have to be that way.
(The Outline also quotes Brad and links to his Death to Bullsh*t project, which demonstrates a fine collection of truly awful ad-driven dark patterns.)
Is your media company struggling with balancing revenue and user experience? We can totally help you with that. Get in touch for a workshop, executive session, or design engagement.