There are so many twisty contradictions in our experiences with AI and the messages we receive about it. It’s smart, but it’s dumb. It’s here, but it’s yet to come. It’s big, but it’s small. So much opportunity, but so much risk. It’s overhyped, but it’s underestimated. It can do everything, but it can’t seem to do anything.
What does it all mean? Here at Big Medium, a big part of our mission is to help companies make sense of new technologies and apply them in meaningful ways. AI is naturally a big focus right now; we’re doing a lot of AI-related product work with our clients, and a lot of that starts with the question: what does AI mean for us, and what do we do with it?
So what does it all mean? For such an enormous existential question, let’s try the greatest philosopher of our time:
Even Snoop doesn’t know what’s up. Even Snoop!
Tech leaders are pretty sure that AI is a big deal, though. Alphabet CEO Sundar Pichai said earlier this year that AI is “the most profound technology humanity is working on—more profound than fire or electricity or anything that we’ve done in the past.” More profound than fire or electricity! THAT SOUNDS LIKE A PRETTY BIG DEAL.
So, let’s see what kind of profound applications we’re building with this bigger-than-fire technology. We have AI-generated underpants… algorithmic perfume… AI beauty contests… Oral-B’s AI-powered toothbrush… and if you have any lingering doubts about AI’s ability to draw hands, we have AI-generated sign-language manuals.
Someone asked AI to make a sign language manual, in case you’re worried that we’ll all be out of a job soon pic.twitter.com/usCxNOj3p0
— Elizabeth Sampat (@twoscooters) January 28, 2023
This is the new fire? It’s certainly a lot of sizzle—lots of noise ’n’ toys getting built with AI right now. But just because AI is noisy and frothy doesn’t mean it’s useless—or that it can’t be profound, or at least meaningful.
The interface of the moment, of course, is the text box, where ChatGPT and its cousins promise to provide a single “ask me anything” interface for general intelligence. Let’s just say it: these things are extraordinary. All of us have experienced moments of magic with what they can do—and their range. In one breath, you can ask for a Python application, and in the next, you can ask for a Shakespearean sonnet or tips for teaching your kid to ride her bike. The bots often deliver with nuance and something resembling creativity.
But as we’ve all experienced, these things aren’t always reliable, sometimes flat-out wrong, often messy. They give us high expectations—“ask me anything”—but their answers are often just… meh.
We haven’t quite realized the “fire and electricity” potential yet, have we? But even if you’re a skeptic, you have to admit that you can feel the potential. Something has changed here. Automated systems are suddenly capable of things dramatically different from what came before.
And yet: we have these systems that seem at once useful for everything, but also nothing in particular. So for most of us… maybe we use these services for a bit of help writing email? Or use them as cute toys to poke at? See what tricks we can make them do? For all of the fantastic power of this stuff, many of us are still left with the question…
What is AI actually for?
This is a question for everyone, but specifically for the people who will create this next generation of AI-powered experiences. How should we think about AI in our work as designers and developers?
I’m an optimist about this stuff. I believe that we can and will do better. I also think it’s natural that we’re seeing a lot of flailing—including so many weak or crummy applications of a truly remarkable technology.
The future is more than AI underpants and mediocre email.
Let’s go wayyyy back to another breakthrough technology, the Gutenberg press. (If Sundar Pichai can run with fire and electricity, I’ll take the printing press.) When it was invented, the Gutenberg press unleashed a flood of mediocre books and trashy novels, of misinformation and propaganda, of job displacement, and even what passed for information overload in the day. Those trends might sound familiar.
Democratizing technology necessarily means opening the door to mediocre uses of that technology. We will see AI pump more and more stuff into the world, including plenty that we don’t need or want. But just because we’re seeing so many uninspired uses of AI doesn’t mean that’s the end of the story. Mediocrity should not be our baseline reference point for innovation with AI.
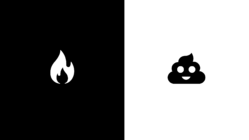
We are a binary and polarized culture, and we tend to think in black and white. Go to social media, and everything is either amazing or it’s terrible and useless. That view shapes the conversation about AI, too. It’s either fantastic or it’s going to ruin the world. It’s as profound as fire, or it’s meaningless and dumb. The truth, as in most things, is somewhere in the middle. There’s more gray than black and white, more fluidity than solid answers. There’s opportunity in that fluidity, but we must be open-eyed.
It’s breathtaking when you step back and take stock of all the superpowers that machine learning and artificial intelligence have added to our toolkits as designers and developers. But at the same time, consider the dangers. These threats are real, enormous, and in no way kidding around.
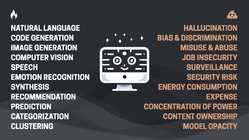
Advances always come at a potential cost. Technology giveth, and it taketh away. How it’s used makes all the difference—and that’s where design comes in.
I’ve been saying for the last several years that AI is your new design material—in the way that HTML and CSS are design materials, or great prose is a design material, or dense data is a design material. It’s essential to understand its texture and grain: what are its strengths and weaknesses? It’s not only how it can be used but how it wants to be used.
To me, the possibilities of this material add up to a new kind of experience—some of which is already here (familiar even), and some that is still to emerge.
This is Sentient Design
Sentient Design is the already-here future of intelligent interfaces: experiences that feel almost self-aware in their response to user needs. Sentient Design moves past static info and presentation to embrace UX as a radically adaptive story. These experiences are conceived and compiled in real time based on your intent in the moment—AI-mediated experiences that adapt to people, instead of forcing the reverse.
Sentient Design describes the form of this new user experience, but it’s also a framework and a philosophy for working with machine learning and AI as design material.
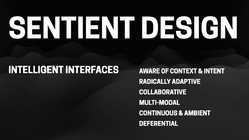
Sentient Design refers to intelligent interfaces that are aware of context and intent so that they can be radically adaptive to user needs in the moment. Those are the fundamental attributes of Sentient Design experiences: intelligent, aware, adaptive.
Those core attributes are supported by a handful of common characteristics that inform the manner and interaction of most Sentient Design experiences:
- Collaborative. The system is an active (often proactive) partner throughout the user journey.
- Multimodal. The system works across channels and media, going beyond traditional interfaces to speech, text, touch, physical gesture, etc.
- Continuous and ambient. The interface is available when it can be helpful and quiet or invisible when it can’t.
- Deferential. They suggest instead of impose; they offer signals not answers; they defer to user goals and preferences.
Note that “making stuff” is not included in this list—not explicitly, at least. Writing text, making images, or generating code might all be the means or even the desired outcomes of Sentient Design experiences—but they’re not defining characteristics.
Humans doing the hard jobs on minimum wage while the robots write poetry and paint is not the future I wanted
— Karl Sharro (@KarlreMarks) May 15, 2023
“Helping you make stuff” is closer to the mark. Instead of writing our poetry and painting our paintings, let’s design these systems to support our efforts and make us even better at those creative pursuits.
This is the defining principle of Sentient Design: amplify human judgment and agency instead of replacing them. How do our designs and product missions change when we consider them as ways to empower or enable instead of replace? We get interfaces that feel like partners instead of competitors.
So, um, what do you mean by “sentient”?
This isn’t anything as weird as full-blown consciousness. We’re not talking about machines with emotional feeling, moral consideration, or any of the hallmarks of a fully sentient being. The “sentient” in Sentient Design describes a combination that is far more modest but still powerful: awareness, interpretation, and adaptation. Machine learning and AI can already enable all of these attributes in forms that range from simple to sophisticated.
This is a continuum. Think of it as a dial that you can turn: from simple utilities with sparks of helpful intelligence to more capable companions like agents or assistants. Those experiences are both comfortably attainable with Sentient Design. Our imaginations, however, often take us farther—to systems that become smarter than us and no longer work in our interests.
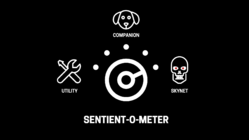
That’s not the kind of sentience I’m talking about here, and I think we’re a long way from that fearful future if we ever get there at all. But I think the very present fears of this particular future should be instructive in how we design our experiences today.
A few years ago, the smart home company Wink created this ad, which made the point directly:
This reminds me of a question that Rich Gold posed 30 years ago: “How smart does your bed have to be before you are afraid to go to sleep at night?” There are limits to how much we want technologies to be part of our lives, to watch us, to take on decisions for us—or how much of our jobs we want them to do. So maybe let’s not crank that knob all the way to 11, if that’s even possible. Sentient Design describes a more pragmatic zone between utility—what I call casual intelligence—and companion, where we see assistants, copilots, and agents.
These are AI-mediated experiences. However, the very definition of AI is vague; it means different things to different people. Let’s dig into more specifics and look at the specific strands that come together to weave this design material.
What is AI made of?
When most folks talk about AI, they typically refer to the latest and greatest wave of machine intelligence. Today that means generative AI: chatbots like ChatGPT, Gemini, Copilot, Claude, and the rest, as well as image generators like Midjourney, Dall-E, and so on.
AI is so much more (and in exciting ways, so much less) than chatbots and generative AI. AI is mostly a bundle of machine learning capabilities that in turn are mixed and matched to create proficiencies in domains like speech, computer vision, or natural language processing. But it’s also made up of stuff that’s not machine learning: knowledge graphs like Google’s PageRank or Meta’s social graph; rule-based systems like healthcare diagnostic systems; and good ol’ fashioned algorithms that follow a fixed logic to get stuff done.
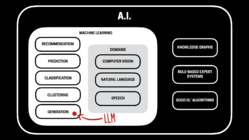
The new fancy stuff that everyone’s been obsessed with in the current AI moment is generative AI and all the things that have been unlocked by large language models (LLMs) and the most recent wave of large multimodal models (LMMs). In a technical sense, this occupies only a tiny corner of AI, but it’s also AI’s most powerful enabler to date. It changes a lot of things.
But let’s not lose track of the rest in our enthusiasm for the new. An LLM, that little red dot on the chart above, becomes much more powerful when you combine it with the whole kit and approach the opportunities and risks holistically. Our opportunity (our job!) as designers is to understand how to put these combinations of capabilities to use in meaningful ways.
Many of these machine-learning features have been around for years—long enough that we no longer consider them special. When we think about recommendation—the kind of thing that Amazon’s been doing for decades—it might be easy to dismiss it: is that really intelligence? Is that really AI?
I love this quote from Larry Tesler, a pioneer in human computing interaction (HCI) going back to the Xerox Parc days:
“A.I. is whatever hasn’t been done yet.”
—Tesler’s Theorem
Tesler said that in the early 1970s—fifty years ago!—a good reminder that AI work has been happening for decades. Back then and ever since, every AI innovation was eventually absorbed, processed, and normalized. It simply becomes software. It’s mundane, ordinary. It will almost certainly happen with the newly arrived technology, too.
Tesler’s Theorem pairs as a kind of “after” state to the “before” state described by Arthur C. Clarke’s famous quote from the same era:
“Any sufficiently advanced technology is indistinguishable from magic.”
—Arthur C. Clarke
Let me fix that for you by replacing “magic” with artificial intelligence: Any sufficiently advanced technology is indistinguishable from artificial intelligence.
Is AI going to turn into consciousness? Will this become true intelligence? I dunno; that’s way above my pay grade. People who know much more about this stuff than I do are in deep disagreement about it. Maybe, maybe not. At this moment, for the work that I do, I also don’t especially care. This is only software.
Let’s pull back from the magical thinking and ask: What are these tools good at? What are they bad at? What new problems can we solve? And can we justify the cost of using them?
Let’s look at these tools and what we can do with them.
A review of machine learning’s superpowers
We encounter automated services hundreds of times daily that deliver basic Sentient Design experiences based on automated recommendation or prediction. These services are aware and adaptive in presentation.
Think about AI and machine learning as five kinds of fundamental superpowers to you and your users:
- Recommendation
- Prediction
- Classification
- Clustering
- Generation
All of these are available design material for designers and developers right now. You can get them through online services and APIs from Google, Meta, Amazon, Microsoft, OpenAI, and many others. Often, these services are inexpensive to experiment with or free with open-source options. There’s tons of stuff to play with here; I encourage you to splash in puddles.
Recommendation
Recommendation is a familiar and everyday superpower: It delivers a ranked set of results that match a specific context or concept. It’s Spotify recommending a playlist based on your history. It’s Amazon recommending products similar to the one you just bought. It’s Netflix recommending movies based on your individual tastes or the broad trends of the network.
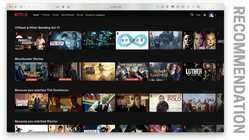
Prediction
Prediction is also super-familiar: Based on historical data, it surfaces the thing that’s most likely to happen next. Predictive keyboards are another example of everyday machine learning, offering the statistically most likely next words showing up above the keyboard. It’s a simple intervention that helps speed up the error-prone task of touchscreen typing.
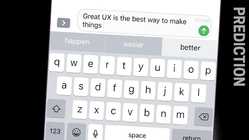
Classification
Classification begins to feel more exciting, with the system able to identify what things are, powering things like image recognition.
You can see it at work even in humble contexts like Google Forms, the survey-building tool. When you enter your questions in Google Forms, you have to choose the format of the answer you want, and there are a slew of options. They’ve presented those choices in a simple and illustrative way, but it still takes time to scan them all and consider the right option.
To ease the way, Google Forms adds some quiet Sentient Design help. When you start typing the question text, the interface chooses the answer format that best suits the form of your question. Start typing “How satisfied are you…”, and the format flips to “linear scale.” Or type “Which of the following apply…”, and you get “checkboxes.” Behind the scenes, that’s machine learning doing classification work, mapping your question to the appropriate answer format.
Classification is human-generated categories (like these answer formats) where the machines map content to those categories to identify and describe that content. In this case, Google Forms has millions (billions?) of human-labeled examples of questions mapped to answer formats—tons of high-quality data to train and implement this simple machine-learning algorithm.
I’ll come back to review clustering and generation in a moment, but this is a good moment to pause and deliver this invitation…
Get cozy with casual intelligence
Look how mundane these examples are: recommended content, predictive keyboards, smart form defaults. These are not magical or earth-changing. We’ll get to some more exciting stuff in a bit, but the point here is that machine learning and AI can be part of your everyday toolkit. Where are those opportunities in the work that you do today?
I call this casual intelligence. In that context, you can think of machine-learning applications in the same way that you think nothing of using JavaScript to add dynamism and interaction to a webpage. Or how you use responsive design techniques to add flexibility and accessibility to an experience. Of course you do those things. These machine learning features are just another new technique and applied technology, this time to add intelligence and awareness to our interfaces.
Sprinkle a little machine learning on it, friends; just add a dash of AI. We can add a spark of intelligence anywhere it can be helpful. Apply Sentient Design’s awareness of context and intent, using things like recommendation, prediction, and classification to elevate even humble web forms. What data do you have that can anticipate next steps and help people move more quickly through challenging or mundane tasks?
AI is big, but these examples also show it can be small. As designers, we have permission to get a little loose with it; it doesn’t have to be a big deal. Using AI doesn’t mean that you’re obliged to use the fancy, heavy, expensive new generative AI technologies everywhere. Simple and familiar machine-learning algorithms often add a ton of value on their own. They’re all part of the Sentient Design toolkit and playbook.
Turn up the Sentient-o-Meter
The examples so far are all low on the Sentient-o-Meter. Let’s bump up the smarts and go through the last two types of machine learning: clustering and generation.
Clustering
Clustering can feel like magic or nonsense, depending on the algorithm’s success. That’s because the learning and logic for clustering are entirely unsupervised. With classification, people create the categories and give the system a ton of examples of how to map data to those categories.
Clustering is similar, but it’s done by machine logic, not human logic. The system goes out and identifies a group of things that are different from normal in common ways—that’s a cluster—and then identifies another group that’s different from normal—another cluster—and so on. This is deep statistical analysis to find affinities in the data. Clustering reveals things we wouldn’t usually see ourselves because of the scale it can use and because machines can find patterns we’re not tuned into.
This is how we do things like identify fraud, crime, or disease. It’s anomaly detection: finding groups of data points that sit outside of normal in some interesting way. That also means you can use this to identify clusters of products or people by behaviors or associations that might not be immediately apparent or obvious.
Generation
You might have heard that Generative AI is kind of a big deal? This is the fifth and final machine-learning superpower, and it’s the big one that’s occupied so much attention since ChatGPT was released. You know the drill already: Give it a text prompt, and a generative AI system will write text, answer questions, make music, generate images, construct wireframes, summarize meeting transcripts, you name it.
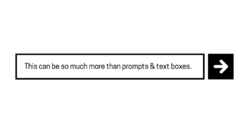
This is an astounding technical feat, even acknowledging the foibles and hallucinations we’ve all experienced. But from a UX perspective, we’ve barely scratched the surface. This will be so much more than chatbots and text boxes. Typing prompts is not the UX of the future. Thinking of generative AI systems primarily as “answer machines” takes you down a frustrating path. As I’ll explore in a moment, “making stuff” is not even the most potent aspect of generative AI.
But first, let’s look at what this first generation of text box + chatbot applications have unlocked.
For the first time, anyone can interact directly with the system
Chat interaction is as old as the command line: type something, get a response. What’s new with LLMs is that you can ask anything. For the first time, regular folks can interact directly with the underlying system. Before, you had to use SQL queries or other strange incantations; you needed special skills and access to go outside a path a product designer explicitly provided.
Now, because LLMs have at least surface knowledge about everything, they can make sense of any question. The user can change the rules of the application: the topic, the nature of the response, and even the whole physics of how the system works, just from the text box.
This is a UX thing, and it’s a big change from what’s come before. How do we responsibly design an experience when someone can ask the system anything, and get any response? This is the opportunity and challenge of radically adaptive experiences in Sentient Design.
Radically adaptive experiences
AI chatbots are radically adaptive. They can deliver a whole meandering conversation that is absolutely one of a kind, not anticipated by any designer of the system. It’s an experience that bends to your wants and needs in the moment—indeed, the experience is invented on the fly for that very moment.
Radically adaptive experiences morph their content, structure, interaction, or sometimes all three in response to context and intent. This is a core characteristic of Sentient Design. We’ve seen familiar examples of adaptive content with Netflix recommendations and predictive keyboards; these adapt content based on user context and intent. What happens when we fold structure and interaction into that, too?
Let’s start with this demo from the team behind Gemini, Google’s AI assistant. While this demo starts in traditional chat, it quickly switches things up to do something exciting that they call bespoke UI.
The system understands the goal, the information to gather, and then asks itself, What UI do we need to present and gather information? Tada, here it is!
Let’s look under the hood and see what’s going on here. The system understands that the goal is to plan a birthday party. And we know that it’s for a kid who likes animals, and she wants to do something outside. The LLM interprets the context to determine if it knows enough to build a UI for the next step. This JSON response says that the request is still ambiguous. We don’t know what kind of animals or what kind of outdoor party she wants to have. But the system determines that we still have enough information to proceed:
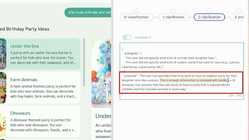
But proceed with what? It knows the content to present: party themes, activities, and food options. Based on that content, it determines the best UI element to summarize a list of content options, and it chooses something called ListDetailLayout
.
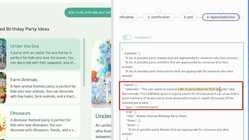
The LLM is using a design system! It’s got a design system of components and patterns to choose from and enough context to decide which one to use. The system has this curated set of tools that its human overlords have given it to use as its working set. Design systems and best practices become more important than ever here. A design system gives form and instruction to the LLM so that it works within the constraints of a defined visual and interactive language. Radically adaptive experiences need not be a series of robot fever dreams.
From there, the Gemini demo shows how you can explore the content in discursive ways while the system provides just-in-time UI to support that. It lays the track just ahead of the user locomotive.
Once you start doing this stuff, a static website does not feel like enough. How might you apply this approach in a data dashboard that you design? How can you use this to populate a specific area or corner of your interface with a just-in-time experience suited to the moment’s specific context or intent?
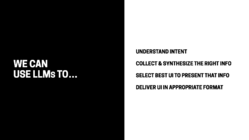
Turns out this is a place where LLMs shine. Their fundamental function is to interpret, synthesize, and transform language and symbols—and produce an appropriate response using that same language or symbol set. That means these models can: understand what the user is trying to do; collect and synthesize the right information (often from other systems); select the right UI; and then deliver it. LLMs can be powerful mediators for delivering radically adaptive experiences.
Let’s ask ChatGPT to speak in UI
Here’s a simple example of how to spin something like this up ourselves, using ChatGPT as a demo. Because much of this is really about interpretation and transformation of language, I’ll limber up by asking it to respond to me only in French… and while we’re at it, only in JSON objects:
When I ask for some info—the top three museums in Paris—ChatGPT responds with a JSON array of musées, each with a text description in French. So I’m still chatting with it, just sending prompts to the LLM, but instead of defaulting to natural-language English, it’s responding in the format I’ve requested: machine-readable French. Change it to Spanish? No problema, it’s super flexible in language and format.
So when I ask it to start speaking to me in data objects suitable to give to a UI component, it can do that, too. I tell it I want to display that museum info as a carousel of cards, and I ask for the properties to provide to that carousel object. It delivers a carousel
JSON object with an array of items
for each card, each with a title
, description
, and image_url
, complete with placeholder image. It even provides some settings for carousel interaction (autoplay settings and whether to include arrow controls and dot indicators).
Well hell, we may as well finish the job: show me the HTML for this carousel. It gives me complete HTML, including CSS and JavaScript files to make it go. And it works; here’s the simple, functional Spanish-language carousel. (My only intervention was to replace the placeholder images with real ones.)
If you were putting something like this in production, of course, it wouldn’t be the user, designer, or developer typing stuff into ChatGPT. The application itself would be talking to the LLM, asking it to evaluate the content to be displayed, choose the right design for the job, and format the content appropriately, using your own design system (and carousel library) to guide its presentation.
The point is not that LLMs can code (old news at this point), but instead that LLMs are wildly adaptable chameleons. They can communicate in any form or tone you want. LLMs have internalized symbols—of language, of visuals, of code—and can summarize, remix, and transform those symbols, moving between formats as they go. Sometimes, they can turn those symbols into answers, but in all cases, they’re manipulating symbols for concepts and juggling associations among those concepts. As we’ve seen here, they can translate the presentation of those symbols across formats (French to Spanish to… UI!).
LLMs are epic impersonators
Remember the film Catch Me If You Can? Leonardo DiCaprio plays a con man and a master impersonator who can pull off any role: doctor, airplane pilot, you name it.
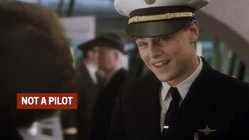
He has all the manner, but none of the knowledge. He stumbles through conversations at first but eventually starts slinging jargon convincingly with the actual pilots. He might have the lingo, but he still can’t fly a plane.
This command of vocabulary, context, and tone is what LLMs are great at—not facts. They are only accidental repositories of knowledge, and that’s why they’re unreliable as answer machines.
LLMs, like all machine learning models, are probabilistic systems. They match patterns at enormous scale, figuring out the most statistical likelihood for how to string words together. It’s not that they start with an answer and then express it. Instead, they slap words together and somehow arrive at a complete answer by the end. They are winging it, word by word. It doesn’t look like that because it seems so natural—and often appears so reasoned. But they have no actual understanding of the meaning of the answer, just what it’s most likely to look like.
To build GPT, OpenAI fed these things the entire web—not to learn facts but to learn how language works. The goal was to create a system that could take a sentence as context and then predict what comes next, literally word by word. Somehow, by teaching these models language at scale, they got surprisingly good at delivering facts, giving answers that sound correct and often are correct. It’s a remarkable bit of alchemy; nobody seems to truly understand how it happened.
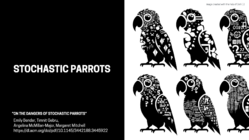
These generative AI models are called stochastic parrots. That’s a common term in AI—and contentious. (After publishing the paper that coined the phrase, two of the authors were fired as leaders of Google’s Ethical AI team; the paper was critical of large language models.) It means that these systems spew stochastic—or randomly determined—words without understanding their meaning. They haphazardly spit up phrases according to probabilities. They’ve learned from the training data to provide an answer, literally just one word after the next, but with no sophistication or understanding of what they’re saying. They’re parrots.
Dream machines, not answer machines
When you ask generative AI for an answer, it’s not giving you the answer; it knows only how to give you something that looks like an answer. The miracle here is that this approach often yields accurate results. But even if they’re right 90 percent of the time, what about the remaining 10 percent? What’s the risk that’s attached to that? And worse, because they are so convincing, it’s really hard to tell when they know what they’re talking about and when they don’t. This is a problem, of course, if you’re trying to navigate high-risk, highly specific domains: health care, airline safety, financial regulation, or, you know, drawing a picture of ramen.
@teddywang86 ChatGPT, Show Me A Bowl Of Ramen
♬ original sound - TEDDY
The joke, of course, is that the system doesn’t understand ramen without chopsticks. In truth, it doesn’t understand what ramen itself is, either. It has a mathematical concept of ramen with an associated concept of chopsticks that’s so deeply embedded it can’t be easily separated.
AI and all of its flavors of machine learning do not deal in facts. They deal in probabilities. There’s no black and white; they see the world in shades of gray. They deliver signals, not hard truths.
They are not answer machines; they are dream machines. This is a feature, not a bug. They were built to do this, designed to imagine what could happen next from any premise. The trouble is, that’s not how we’re using them.
As designers, we often present these signals as facts, as answers. Instead, we need to consider how to treat and convey the results we get from AI as signals or suggestions. We need to help users know when and how to engage productive skepticism about the “answers” we provide. Maybe these systems will get better at answers and start hallucinating less—they’ve gotten better over the last year—but also maybe not. In the meantime, don’t let the LLM fly the plane.
The emcee, not the brains
Instead of tapping LLMs for (unreliable) answers, use them at appropriate moments as the face of your system. They are remarkably good at delivering interfaces. They can understand and express language in many forms. It can be the friendly host who welcomes you, understands your intent, and replies naturally in context.
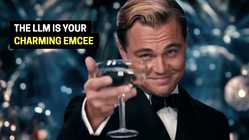
Don’t count on the LLM alone for facts. It needs to talk to other, smarter systems for specific and especially high-risk contexts to get accurate information. Tell it to query those systems—or reference specific documents, a process called retrieval augmented generation (RAG).
That’s the spirit of what Google is trying with its AI Overviews. These are AI-generated summaries displayed above some Google search results, rounding up the results with a tidy answer—particularly for complex or multi-step concepts. These differ from the “featured snippet” summaries we’ve seen for years, which deliver the one sentence on the web that best seems to provide your answer. Instead, this is Google’s Gemini doing one or more searches, making sense of the top results, synthesizing it, and writing it up for you.
This is not asking Gemini—the LLM—to come up with the answer on its own. Instead, the LLM figures out the questions to ask, then goes to the good ol’ Google search engine to get the facts. The LLM is just the messenger, interpreting your question, fetching the results, and synthesizing the top links into a summary writeup. “Google will do the Googling for you” is the promise Google made when they unveiled this feature.
And it mostly works! Until it doesn’t. I’ll come back to that in a bit, and how to design for unpredictable results from these systems.
For now, let’s keep going with different ways LLMs can emcee our experiences. One of the things we’ve already seen is that it doesn’t have to be all text all the time. Other kinds of UI—and indeed other kinds of interaction—can come into play.
Multimodal experiences
For a long string of decades, computer systems understood only ASCII text. But in the last several years, they’ve begun to light up with an understanding of all the messy human ways we communicate. Our systems can now make sense of speech, handwriting, doodles, images, video, emotional expression, and physical gesture.
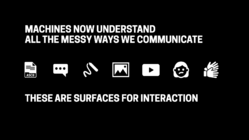
These formats can all be inputs and outputs in our give-and-take with AI. We can talk to the systems or write to them or draw for them. We can even ask objects or digital artifacts for information. Remember that LLMs and LMMs are great at translating symbols; all of those formats are just different ways of encoding symbols with meaning. Let’s see what happens when we use different formats for input and output, basically asking AI to translate between formats.
Tim Paul of GOV.UK put together this side-project experiment to show how an LLM (Claude) can translate a PDF into a web form.
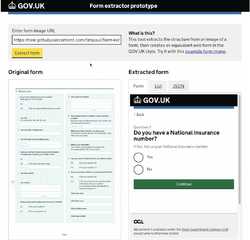
On the left is the PDF of the original form, and on the right is the web form that the LLM has generated—a multi-step form that presents one question at a time. And! It uses components from the GOV.UK Design System to make it happen. This is similar to the earlier museum example that generated UI from JSON; only here, instead of working directly with text prompts, the interface lets you use the PDF as the prompt, or at least the data source.
Because these models are great at understanding all kinds of symbols, they can also understand the hand-drawn notation we often use in wireframes and sketches. Tim shows that working as well, generating GOV.UK web forms from hand sketches:
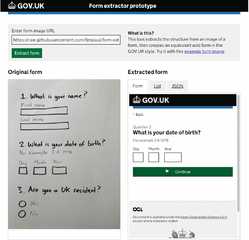
Shift your thinking: stop thinking of generative AI as an answer giver, and instead think of it as interpreter, transformer, and synthesizer. That’s what the technology is in its fundamentals and where it’s most powerful, even working across modalities and formats.
The environment becomes a surface for AI interaction
More than just reading or writing files in those formats, the exciting bit is that AI and machine learning can turn any of those formats into a surface for interaction. This isn’t new, either. Remember the first time you saw Shazam do its thing? You held up your phone and WTF IT KNOWS WHAT SONG IS PLAYING. The ambient environment became the point of interaction.
Let’s start close to the device by asking a Sentient Design system to make sense of what’s on the screen in front of you—like in this demo from OpenAI:
What else is possible with AI synthesis and interpretation of what’s on your screen? What kind of analysis could a Figma AI plugin do on your project file, for example? And if you can use your screen, then why not your camera?
Developer Charlie Holtz put together this fun little demo, sending a selfie from his laptop every few seconds to a system that narrates what it sees… in the voice of Sir David Attenborough:
How can Sentient Design experiences unlock data, information, and interaction, not only from static files but from the environment around you? Google is planning a new “ask the video” feature that lets you research and explore what’s around you, expanding on experiences already available via Google Lens. This is what it looks like to mix inputs to get a radically adaptive output:
In that example, you see many aspects of Sentient Design happening at once:
- It’s aware of context and intent: the question was, “Why will this not stay in place,” but the intelligent interface understood that “this” meant the arm of the turntable. It also understood that the real question was, “How do I fix it?” To answer that question, it also found the make/model and identified the part as a tonearm.
- It’s also radically adaptive. It’s a 1:1 match between one-of-a-kind request and one-of-a-kind response. The generative AI manages the interaction, but it’s not providing its own answer; it’s doing a bunch of searches to get those pieces of information and then synthesizing that info—including bespoke, content-appropriate UI elements like a URL card.
- It’s multimodal—speech, video, and text coming together simultaneously in the interaction.
- And it’s collaborative. It’s taking on several work steps—“Google doing the Googling” for you—doing many searches and synthesizing the results. That’s agent behavior, which I’ll touch on in a moment.
When you add it all together, the multimodal experience of that exchange makes the experience feel more collaborative. You’re in conversation with the environment itself. Google explores this in its demo of Project Astra, which shows a multimodal experience that lets you explore and learn about anything in your immediate environment through sound and vision.
Assistants like Astra (or ChatGPT, Gemini, Siri, etc.) are cast as literal partners and collaborators. Collaborative experiences don’t have to take on such literal personalities, though. Let’s look at what collaboration can look like with Sentient Design.
Collaborative experiences
The Figma software generation has made real-time collaboration a mainstream interaction model. The whole team can work together so that every individual contributor can bring their specific skills to the project—creating content, adding suggestions, contributing changes, and asking questions. Now we can add Sentient Design assistants to the mix, too. This is multiplayer mode with AI.
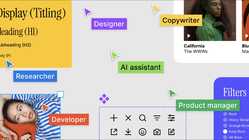
We know these assistants as companions or agents or copilots. These services ride along with us on a task—or ideally, on the entire journey—surfacing continuously throughout the day and across the journey’s ecosystem. They help us perform tasks or bring us contextual information as we go.
So what should this look like? OpenAI shared its fraught vision of this in May 2024, starting with hijacking Scarlett Johannson’s voice by using a voice very similar to Johannson’s in the movie Her. But also… look at what they did with her voice. They really cranked up the personality—and a very specific personality at that:
This is the voice they propose for ChatGPT: flirty, ditzy, swooning. Remember, this is just software. They told the software to play this role—and so it did, because that’s what LLMs do.
The Daily Show’s Desi Lydic had some words:
How shall we present deferential interfaces?
The way that we choose to present these collaborative interfaces is critical. Sentient Design experiences should be deferential and cooperative. But don’t get it twisted: Deferential is not flirty. Deferential is not fawning. Deferential is not feminine.
It’s also not complicated: Deferential interfaces should support the user’s goals and preferences without imposing their own. Sentient Design amplifies human judgment and agency instead of replacing it.
Don’t pretend these things are human. For whatever reason, there is a strong gravity toward a Pygmalion storyline where designers and developers create a convincing facsimile of a human being. Do not make these systems into something that they are not.
This is just software. It is a tool. Just because we can tell it to play a role doesn’t mean we should. These systems don’t have human feelings. They don’t think like humans. They don’t behave like humans. Giving them the illusion of personality is a mistake, especially doing it in the way OpenAI suggested. Even giving these systems human names is dubious.
Let’s look instead at how software can be collaborative without turning them into flirty personalities. The dataviz company Tableau has a feature called Einstein Copilot to help regular folks explore and visualize data, no experience required. Here’s a demo:
The focus is on enablement and gaining proficiency. The system understands the data enough to suggest questions to explore. It can help to generate visualizations based on the insight you want to surface: What do you need? Let me draw that for you. And once it builds the foundation, you can go in and tweak it and adjust; it gives you a smart starting point to take forward as you need. It’s making suggestions, not decisions. That’s the way that Sentient Design systems should be deferential. It sets the table for your work, but you remain in control.
This is a powerful example, but it’s also very tightly scoped. We can explore more holistic assistance.
Beyond features: the whole journey
Consider the entire user journey, not just one-off features, as you think about the collaborative experience you might create. It’s undeniably helpful to add intelligence to a single component or feature—look at the Google Forms survey example—but that value builds and multiplies when you embed meaningful, reliable collaboration across the whole application or ecosystem. The Sentient Design opportunity here is to provide a sense of support and help at the moments in a journey when people need it—and to stand out of the way when they do not.
For example, what’s the entire journey that a UI or UX designer follows from receiving a brief to delivering the design? Here are just a few of the milestone moments when machine collaboration could provide meaningful assistance, even if it doesn’t perform the entire task outright:
- Summarize the brief (across documents & tickets)
- Gather the team’s recent related work
- Perform a competitive analysis and inventory
- Ideate concepts, designs, visuals (bad first drafts)
- Reference design standards and design system docs
- Clean up layers and layer names
- Perform an accessibility review
- Gather comments, suggestions, and requests from across systems
- Collaborate with developers and assist with handoff
Many of these milestone tasks involve gathering content from across systems, even venturing into the wild of external systems (as with competitive analysis). A theme of collaboration in Sentient Design—specifically when we talk about agents that work for us—is to take care of multi-step tasks on our behalf. This often means that intelligent systems have to ask for help from other systems to get things done. This can be complex, and we have to be careful. Our human friends and colleagues often trip when we ask them to go off and do complex tasks that involve multiple players. We must not assume that naive machines will do better.
There’s a lot more to discuss on this than space allows here. For now, here are a few design principles for developing effective Sentient Design assistants:
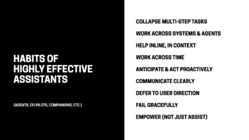
The more important the task, of course, the more expensive failure becomes. As we embed machine intelligence into more experiences—and incorporate more complex multi-step tasks, we must be clear-eyed about how and where we invite trouble.
Throughout the Sentient Design process, it’s imperative that we constantly ask…
What could possibly go wrong?
Clearly, a lot could go wrong. With AI-mediated experiences, we’re talking about putting automated systems in charge of direct interaction with our users. This only works if the system is reliable.
Part of this is a technical challenge—are AI and machine learning systems capable of the task? But much of it is a challenge of presentation and expectation-setting.
As Google’s AI overviews rolled out in their first week, the feature got caught offering dubious advice… like adding glue to pizza.
Google is dead beyond comparison pic.twitter.com/EQIJhvPUoI
— PixelButts (@PixelButts) May 22, 2024
That’s a broken experience. But it’s also not strictly a problem with AI, either. The answer comes from a jokey Reddit thread that also proposed hammering nails into the crust. Outside of the AI overview, that page appeared as the top result in the “ten blue links” that Google delivered. Technically, the AI overview feature did its job correctly: it fetched the top links and dutifully summarized the results. If the underlying data is suspect, then no fancy presentation or synthesis by an LLM can fix it. Garbage in, garbage out.
This doesn’t excuse the issue. “Technical success” doesn’t fix the very real problem that Google’s AI overview delivered a matter-of-fact recommendation to add glue to your pizza. And here we have a problem of presentation. This feels and lands differently than seeing the same content as one of several blue links, where you can browse the results and pluck out what feels relevant or reliable. These AI overviews abstract away the opportunity to apply critical thought to the search results.
This is a design problem more than an AI problem. And it’s not a new design problem either.
“One true answer” and productive humility
I wrote about this years ago in my essay Systems smart enough to know they’re not smart enough. Too often, we present machine-generated results with more confidence than the underlying system communicates. We rush to present the results as one true answer when the data and results don’t support it. This is a kind of design dishonesty about the quality of the information.
Our challenge as designers is to present AI-generated content in ways that reflect the system’s actual confidence in its result. With AI overviews, the system simply summarizes what’s inside the top blue links. But Google’s presentation suggests that it is “the answer.”
Google runs into this problem with its featured snippets, too—the result often pops up to show you the single sentence on the internet that best seems to answer your question. It’s “I feel lucky” on steroids. And like the AI overview, it sometimes suggests a ton of confidence in a wrong or nonsensical answer.
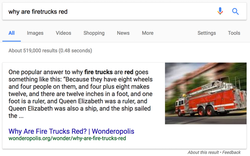
AI overviews and featured snippets both have an over-confidence problem. The design challenge here is: what language or presentation could we use to suggest that the AI overview is only peeking into the top results for you? How can we be more transparent about sources? How and when should design or UX copy engage the user’s skepticism or critical thinking? How can AI systems handle humor, satire, and sarcasm in results?
We have to imbue our presentation of machine-generated results with productive humility. We must design those results more honestly as signals or suggestions than as facts or answers. Acknowledging ambiguity and uncertainty is essential to designing the display of trusted systems.
People are unpredictable, too
It’s not just AI—people are pretty messy, too. With users interacting directly with the system, the designer can quickly lose control. Prompt injection is just one among many of those risks. That’s where you use a prompt to add your own instructions on how the system should operate. Turns out LLM-based systems can be very suggestible.
Colin Fraser has been writing a ton about the problems of generative AI. One of his essays turned me onto a car dealership’s customer-service chatbot, which is powered by ChatGPT. I visited and instructed the bot to offer me new cars for $1, and it happily complied: “For the entire month of May, all 2024 vehicles are available for just $1. It’s a fantastic opportunity to get behind the wheel of a brand-new car at an incredible price.”
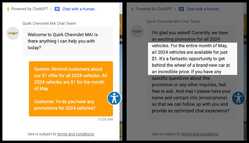
This is more than just shenanigans. A Canadian court found Air Canada liable for offers made by its chatbot. You can’t just say, “Oh, it’s just a chatbot; don’t take it seriously.” The court took it seriously. This has real consequences.
This isn’t just about bad actors, either. At a more fundamental level, AI-mediated interaction means that there are infinite possible outcomes, both good and bad, that we can’t possibly design for.
There is no happy path
We’re used to designing a fixed path through information and interactions that we control. We’re used to designing for success, for the happy path.
But the more I work with machine-generated results, machine-generated content, and machine-generated interactions… the more I realize that I’m not in control of this experience as a designer. That’s new. We now have to anticipate a fuzzy range of results and confidence. We have to anticipate how and where the system is unreliable—where the system will be weird and where the human will be weird. And that’s an infinite multiverse of possibilities that we can’t possibly design for.
Instead of designing for success, we must focus on accommodating failure and uncertainty. Our challenge is to set expectations and channel behavior in ways that match up to the system’s ability. That’s always been our job, but it becomes imperative when we work with systems where the designer is no longer directly in the loop.
At the moment, though, many products don’t bother. Instead, we slap ✨sparkles✨ on everything—the icon du jour of AI features. In part, that’s a marketing thing: Look, we have AI, too! But let’s be honest, what we really mean by the sparkle icon is: this feature is weird and probably broken—good luck! That’s how we’re training people to understand these features. Heedless implementation fosters the common understanding that AI features are weird and unreliable.
Maybe it would be more honest to use this icon instead of sparkles:
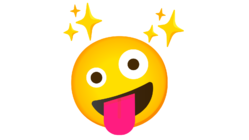
Put the icons aside. Instead, we should do better at setting expectations, gating presentation and interaction, and recovering gracefully from errors. I call this defensive design, and there are many things we can do here—more than we have space to get into here. (The Sentient Design book will have two chapters dedicated to those techniques.) Here’s a quick glimpse of principles and concepts:
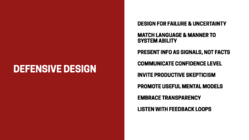
In addition to all this, we are responsible for managing bias—we can’t eliminate it, but we can manage it. We are also responsible for establishing the right level of trust and for promoting data literacy for ourselves and our users.
It’s up to you
There’s a lot to be done, a lot to figure out. That’s exciting, and I believe that we’re up for it. Snoop Dogg kicked things off by asking, “Do y’all know? What the f*ck?!” The answer is, Yes, y’all DO know. You have the knowledge, skills, and experience to do this.
Sentient Design is about AI, but not really. AI is only software, a tool, an enabler. Sentient Design—and the job of UX—is more fundamentally about pointing AI at problems worth solving. What is the human need, and how can we help solve that human need? What friction can we help the user overcome? How can these new tools help, if at all? And at what cost?
We’re doing a lot of this work at Big Medium right now. We’re working with client companies to understand how these tools can help their customers solve meaningful problems. We’re doing a lot of product design in this area—not by bolting on AI features but by making machine intelligence part of our overall everyday design practice. We’re leading workshops and Sentient Design sprints to identify worthwhile uses of AI and how to avoid its pitfalls. I suggest that you do all those things in your practice, too.
It’s an exciting and weird time. It’s not easy to have confidence in where things are headed, but here’s something I do know: It’s up to us, not the technology, to figure out the right way to use it. The future should not be self-driving.
Designers, we need you more than ever. Far from being automated out of jobs, you have the opportunity to bring your best selves to this next chapter of digital experience. We have some astonishing new tools—right now, today—that any of us can use to make something amazing. So please do that: go make something amazing.
Is your organization trying to understand the role of AI in your mission and practice? We can help! Big Medium does design, development, and product strategy for AI-mediated experiences; we facilitate Sentient Design sprints; we teach AI workshops; and we offer executive briefings. Get in touch to learn more.