Jorge Arango explores what it means for machine intelligence to be “used well” and, in particular, questions the current fascination with general-purpose, open-ended chat interfaces.
There are obvious challenges here. For one, this is the first time we’ve interacted with systems that match our linguistic abilities while lacking other attributes of intelligence: consciousness, theory of mind, pride, shame, common sense, etc. AIs’ eloquence tricks us into accepting their output when we have no competence to do so.
The AI-written contract may be better than a human-written one. But can you trust it? After all, if you’re not a lawyer, you don’t know what you don’t know. And the fact that the AI contract looks so similar to a human one makes it easy for you to take its provenance for granted. That is, the better the outcome looks to your non-specialist eyes, the more likely you are to give up your agency.
Another challenge is that ChatGPT’s success has driven many people to equate AIs with chatbots. As a result, the current default approach to adding AI to products entails awkwardly grafting chat onto existing experiences, either for augmenting search (possibly good) or replacing human service agents (generally bad.)
But these ‘chatbot‘ scenarios only cover a portion of the possibility space — and not even the most interesting one.
I’m grateful for the call to action to think beyond chat and general-purpose, open-ended interfaces. Those have their place, but there’s so much more to explore here.
The popular imagination has equated intelligence with convincing conversation since Alan Turing proposed his “imitation game” in 1950. The concept is simple: if a system can fool you into thinking you’re talking to a human, it can be considered intelligent. For the better part of a century, the Turing Test has shaped popular expectations of machine intelligence from science fiction to Silicon Valley. Chat is an interaction cliché for AI that we have to escape (or at least question), but it has a powerful gravitational force. “Speaks well = thinks well” is a hard perception to break. We fall for it with people, too.
The “AI can make mistakes” labels don’t cut it.
Given the outsized trust we have in systems that speak so confidently, designers have a big challenge when crafting intelligent interfaces: how can you engage the user’s agency and judgment when the answer is not actually as confident as the LLM delivers it? Communicating the accuracy/confidence of results is a design job. The “AI can make mistakes” labels don’t cut it.
This isn’t a new challenge. I’ve been writing about systems smart enough to know they’re not smart enough for years. But the problem gets steeper as the systems appear outwardly smarter and lull us into false confidence.
Jorge’s 2x2 matrix of AI control vs AI accuracy is a helpful tool to at least consider the risks as you explore solutions.
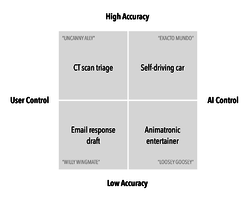
This is a tricky time. It’s natural to seek grounding in times of change, which can cause us to cling too tightly to assumptions or established patterns. Loyalty to the long-held idea that conflates conversation with intelligence is doing a disservice. Conversation between human and machine doesn’t have to mean literal dialogue. Let’s be far more expansive in what we consider “chat” and unpack the broad forms these interactions can take.